Construction and demolition generate 40–50% of the world's waste. From circular economy's point of view, it’s essential that material from this waste can be reused rather than ending up in landfills. Artificial intelligence (AI) helps to automatically screen waste streams and improves the recycling rate of construction.
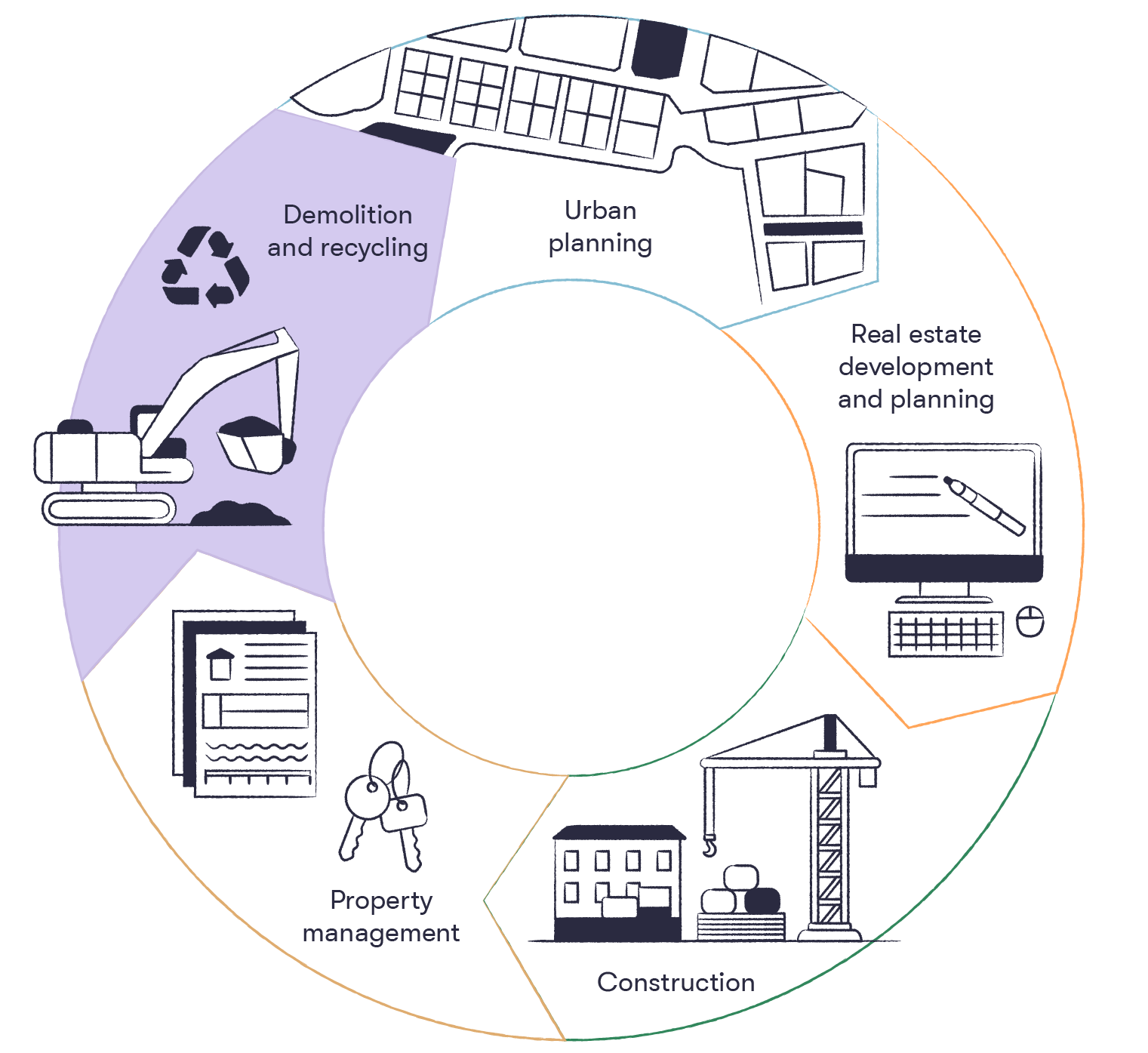
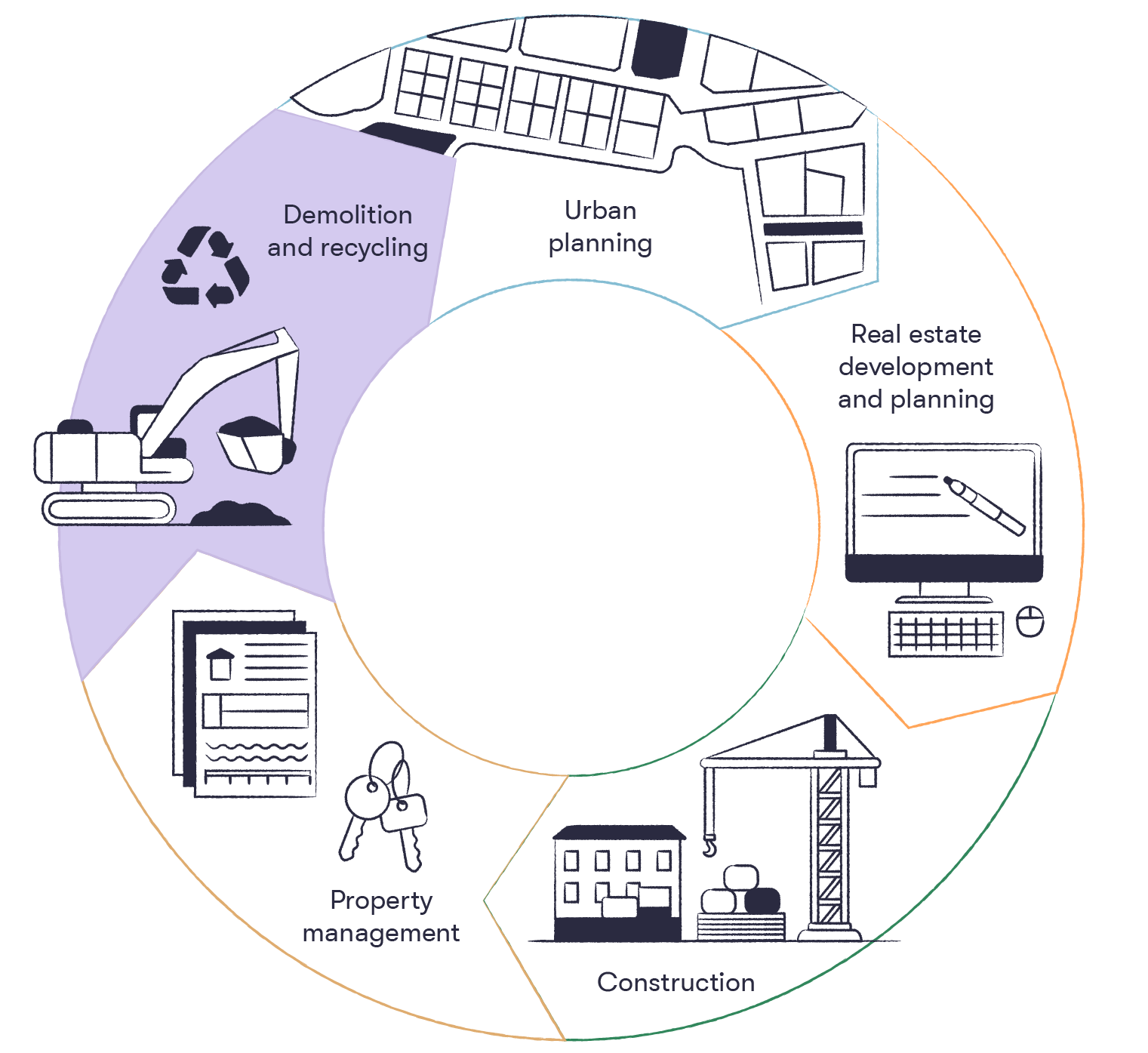
ZenRobotics – robotic waste sorting and recycling
Founded in 2007, ZenRobotics develops intelligent recycling robotics. It's the first company to apply AI-based robots to the demanding task of waste sorting.
Machine learning at the heart of the company
The trajectory of ZenRobotics is interesting. In the beginning, the company built robots and machines, then software, and then total solutions. Now their developers’ focus is shifting again, toward sensors, sensor data, and process development.
The company's best-known product is a robotic waste sorting system, which has been sold to the Netherlands, Sweden, Switzerland, and the United States, among others.
The development work is carried out by a team of about 30 people, most of whom focus on software and automation, and in particular on the development of two waste sorting robots – “Heavy Picker” and “Fast Picker”.
These sorting robots can separate several types of waste with the help of different kinds of sensors, robot arms, and AI. Both robots work on the same principle: waste moves on a conveyor belt, where sensors and cameras allow the system to identify the material for the robot to pick up.
As the name implies, Heavy Picker is stronger and equipped with more versatile sensors: an MIR sensor (infrared), a spectral camera (light), a metal sensor, and a 3D sensor (shape), among others.
Fast Picker works slightly differently – the goal is to do as much as possible with a simpler device. As a result, there are fewer sensors and an RGB camera that detects the material's colors.
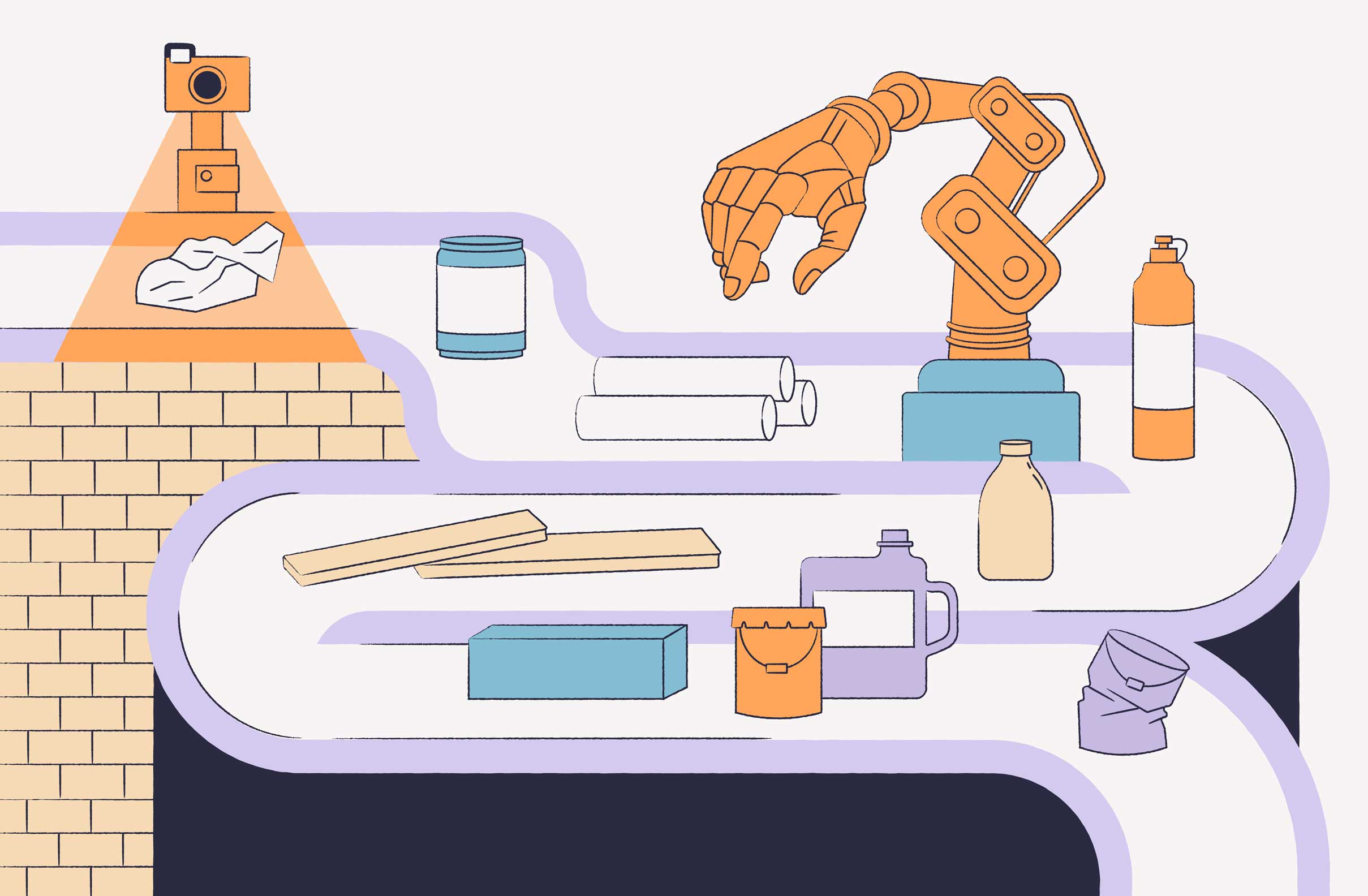
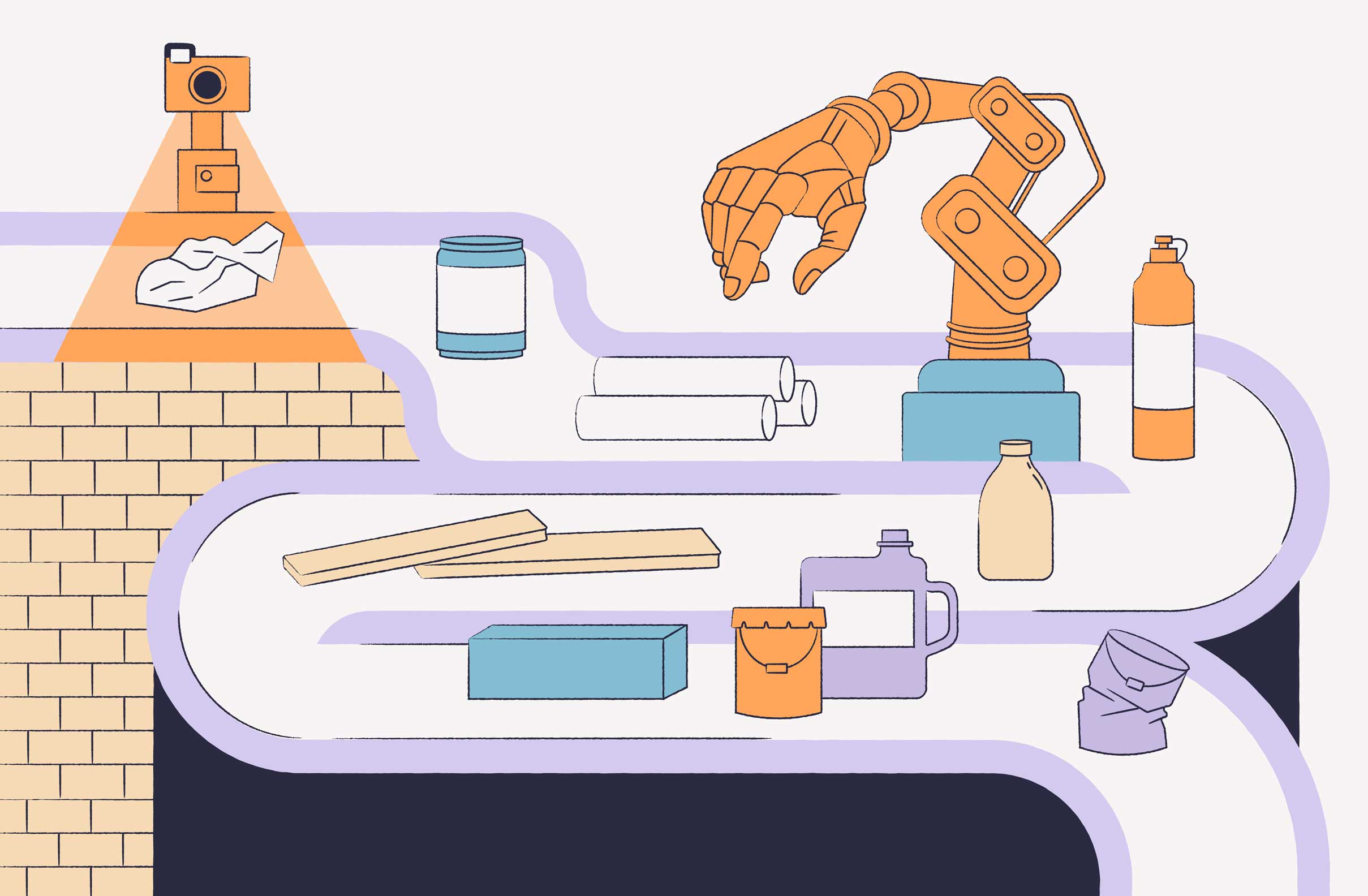
Optimized robotics
In addition to material identification, the other key issue is which materials the robot picks up from the conveyor belt. After identification, the robot processes and evaluates the materials according to a pre-trained model, and it sometimes ignores less valuable materials to ensure it has time to pick up the more valuable ones.
After identifying the material, the robot moves to the right place at the right time. The control is adaptive, meaning the robot can automatically calculate its trajectory so it’s in the right place on the conveyor belt. The goal is to minimize movement so the robot has time to pick up as much as possible.
Ready-made models and further training of robots
In its own development work, ZenRobotics uses as many pre-trained and ready-made machine learning models as possible. Users still need to further train the models onsite.
For example, training data for a material identification model isn't annotated in detail (for example, “PET Bottle, Coca-Cola Zero”), but the model is taught on a material-by-material basis. If the same kind of material in different shapes (for example, different shapes of Coca-Cola Zero bottles) is run on the conveyor belt, the machine learns each object as a separate material.
Generative AI can be used to synthesize diverse training data for material identification models, creating a vast array of images or sensor data of different materials under various conditions. This could dramatically improve the model's accuracy and robustness, enabling the identification of a wider range of materials with higher precision.
For construction and demolition waste, which varies greatly, generative AI can create synthetic data sets that represent the unique characteristics of waste from different sites. This would facilitate the training of robots for specific projects, enhancing their sorting effectiveness and adaptability to different types of waste.
Aha!
Especially in construction and demolition, all waste streams and treatment centers are individual, which is why robots must always be trained on site.
What’s possible with machine vision
Material identification machine vision is constantly evolving, but there's still a long way to go before it works seamlessly. A distant, perhaps unrealistic goal is a universal model that would recognize objects and materials from an almost infinite number of options.
The development of machine vision in recent years has been based on advances in models and algorithms and the technologies behind them. However, currently available technology isn't enough to significantly improve the performance of AI-based models. This means companies shouldn’t try to develop their own advanced image recognition solution, but instead focus on making better use of the available data.
Digitalization of waste management
There is huge potential in the digitalization of waste treatment processes. Megatrends and a tightened regulatory environment are creating positive pressure to reform waste management.
Utilization of data generated in the process is still in its infancy. Sorting plants generally don't have information on the content of incoming and outgoing materials. ZenRobotics' sorting robot provides new types of information for waste processing plants. It detects the quality of incoming waste in real time and automatically notifies the user of any exceptional situations. In this way it produces the information required to implement a circular economy.
Sign up to solve exercises
Ramboll – area reuse planning with Galago
Galago is a machine vision solution for monitoring environment. It's used mainly for monitoring large areas of land, as well as for restoring and rehabilitating contaminated land. Galago identifies problem areas and assists in correcting them and fulfilling stakeholder obligations. The solution uses satellite, drone, and aerial imagery combined with AI for situational awareness across a large area. Based on the image data, the platform provides landowners with recommendations for action. The solution has mostly been used for restoring large mining sites, monitoring the development of areas of forest, nature, and vegetation, and identifying alien and harmful species in large areas.
AI helps the experts
Galago is stepping up on-the-spot inspections, and in some cases even completely replacing them. This saves costs and helps allocate limited inspection resources efficiently. An alternative to Galago is much more expensive human-based monitoring and on-site inspection. In large areas, this means data collection is based on random sampling, which may result in something relevant going unnoticed.
Galago is a great example of an AI solution replacing routine human data processing. It doesn’t completely eliminate the work people do, but frees up expert time for more valuable tasks. Galago’s technology helps landowners make data-driven decisions that save them time and money through automated reporting.
Best practices
The best practices from the practical examples described above can be summarized as follows:
Bringing AI solutions into the production environment is challenging, and maintaining machine learning models often requires significantly more resources than traditional software solutions.
The right level of documentation reduces the need for extra meetings. The documentation can be used to communicate the project's starting points, dependencies, and goals at a glance.
Documentation is also a way to manage expectations by drawing attention to the limitations of AI projects. Otherwise, enthusiasm in an organization for introducing AI may subside if the expectations for the project aren't met by the end result.
Scaling AI functions across an organization takes time. To support change, change agents who understand both the potential of the technology and business processes are needed.
Communicating about AI is only 20% about the technology. It's mostly about the answers to the questions “what”, “how”, and “why”. Simple communication and practical examples work best.
Scaling any technology is a sales task. The goal is to create models in which demand gradually increases and the centralized expertise of the initial phase can be implemented throughout the organization.
In the second chapter, you learned about the following:
Our condensed real estate and construction value chain, including urban planning, real estate development and design, construction, property maintenance, and demolition and recycling
The application of AI, with the following examples: land use planning, environmental monitoring, traffic planning, optimizing energy consumption of buildings, construction site phase identification, onsite communication, apartment sales, business premises rental, customer-oriented optimization of work tasks as part of ERP, preventive maintenance, and waste management
AI solutions are most successful when they serve a clear business need and build on existing processes; on the other hand, AI can create entirely new services or products that complement or challenge existing practices
The same basic solution can serve multiple needs and help achieve different types of goals at the same time, such as operational efficiency and sustainable development goals
Business examples demonstrating that the introduction of AI in companies should be thought of as a continuous learning process rather than a discrete investment