Although AI is a technology, its development and use are activities that require changes in human behavior. The challenges related to using AI are therefore the same as with any other organizational development and change management processes.
The three pillars of AI
1. Business orientation – AI applications need to solve real business problems.
2. Competence – competence in the organization must be sufficient for the development of AI solutions.
3. Data – data must serve a purpose, be of high quality, and be systematically maintained.
In order for the three pillars to support each other and work together, the right kind of organization and interaction are also required.
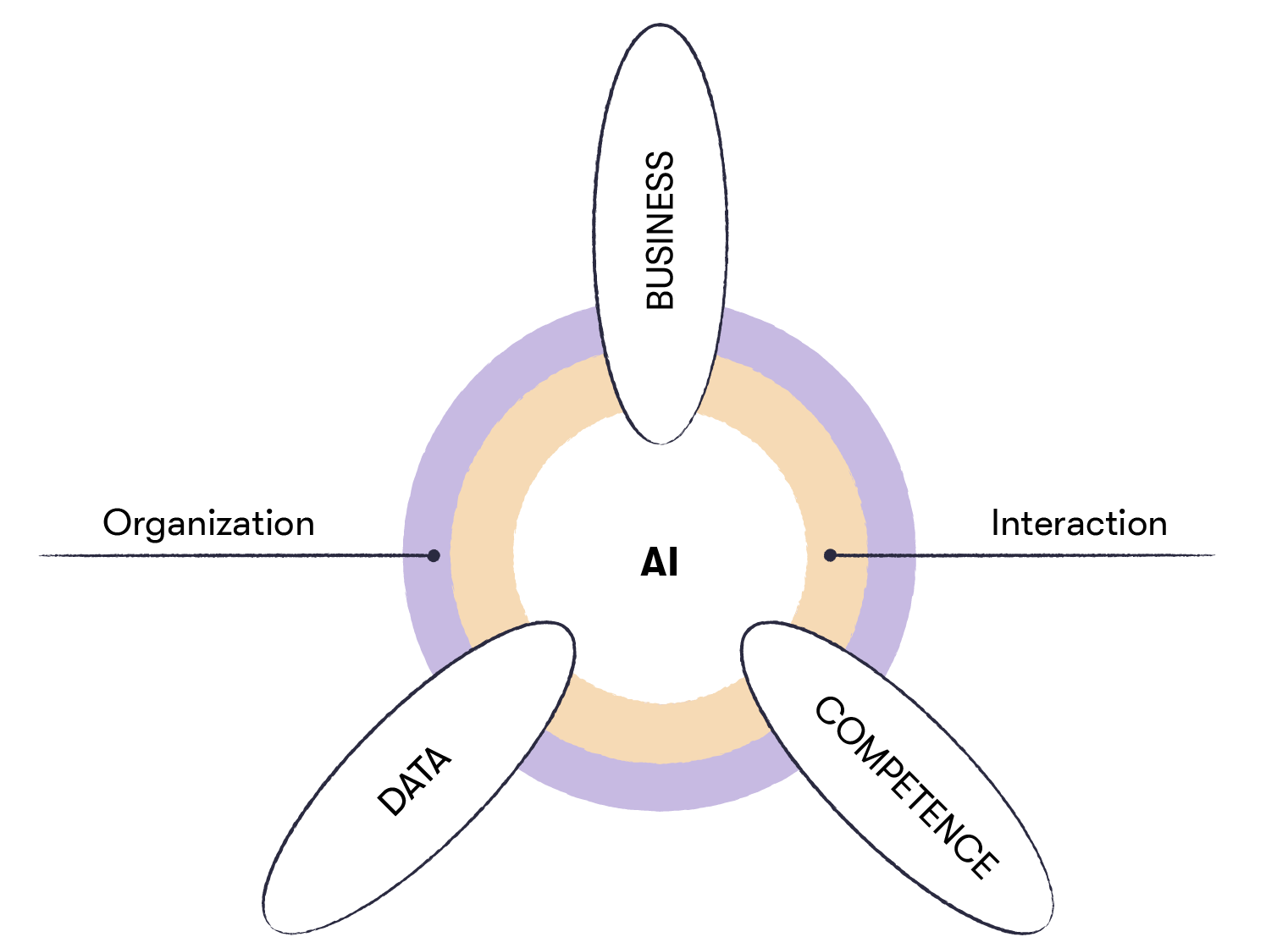
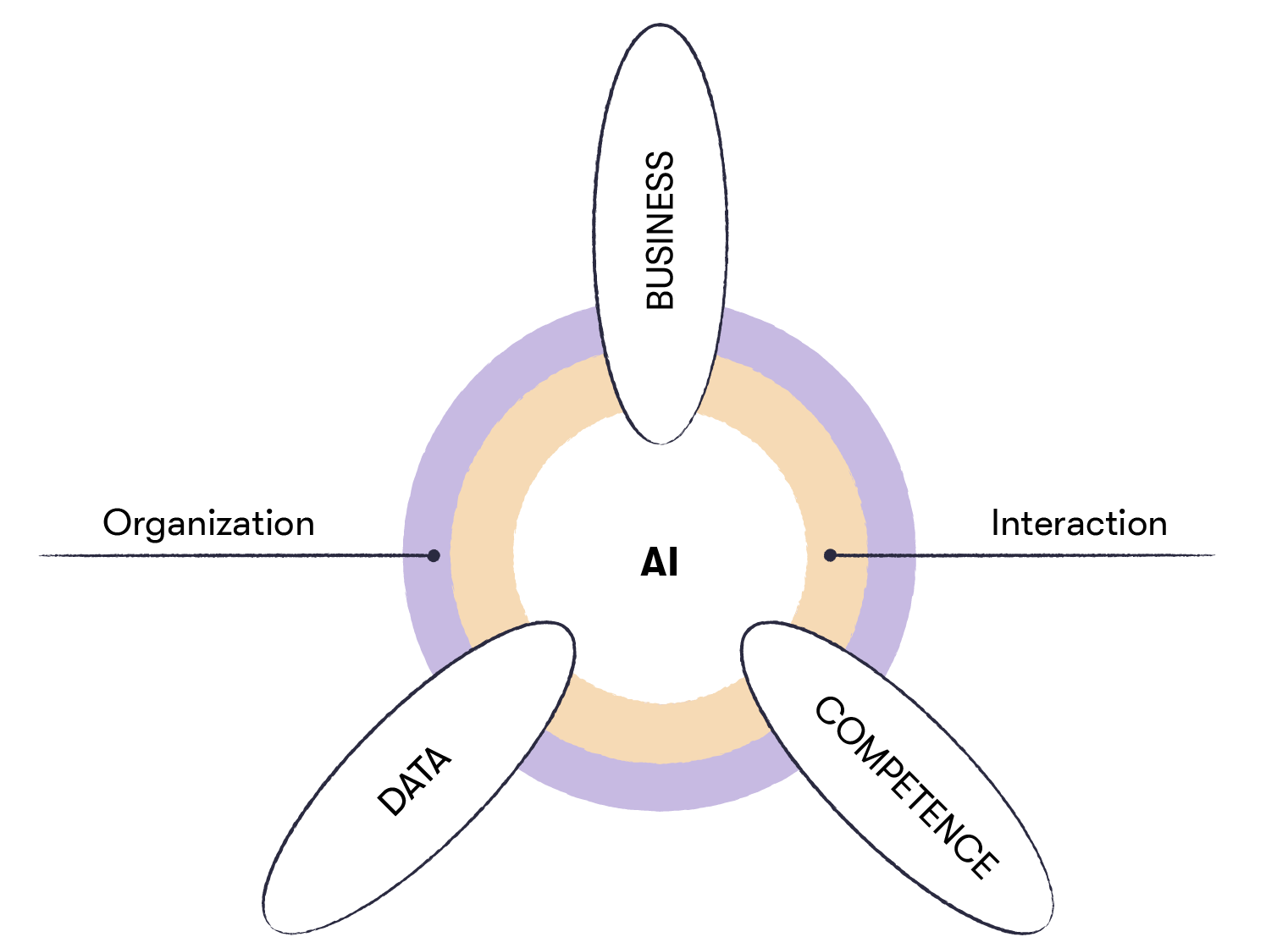
Prerequisites for utilizing artificial intelligence in organizations
In this section, we’ll examine the challenges related to organizations and interaction. The three pillars from the picture above – business, competence, and data – will be covered in Chapter 3.
The organization
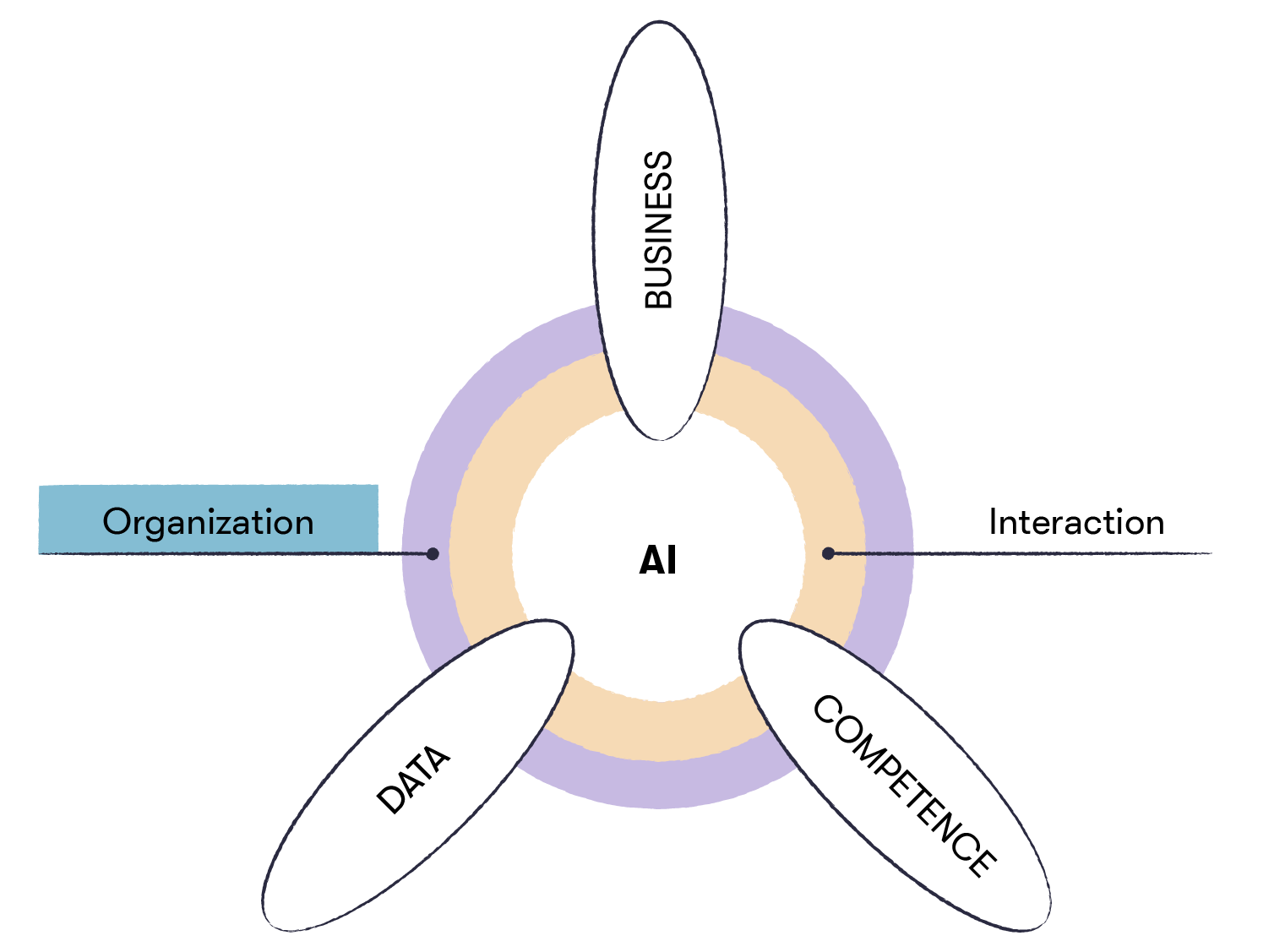
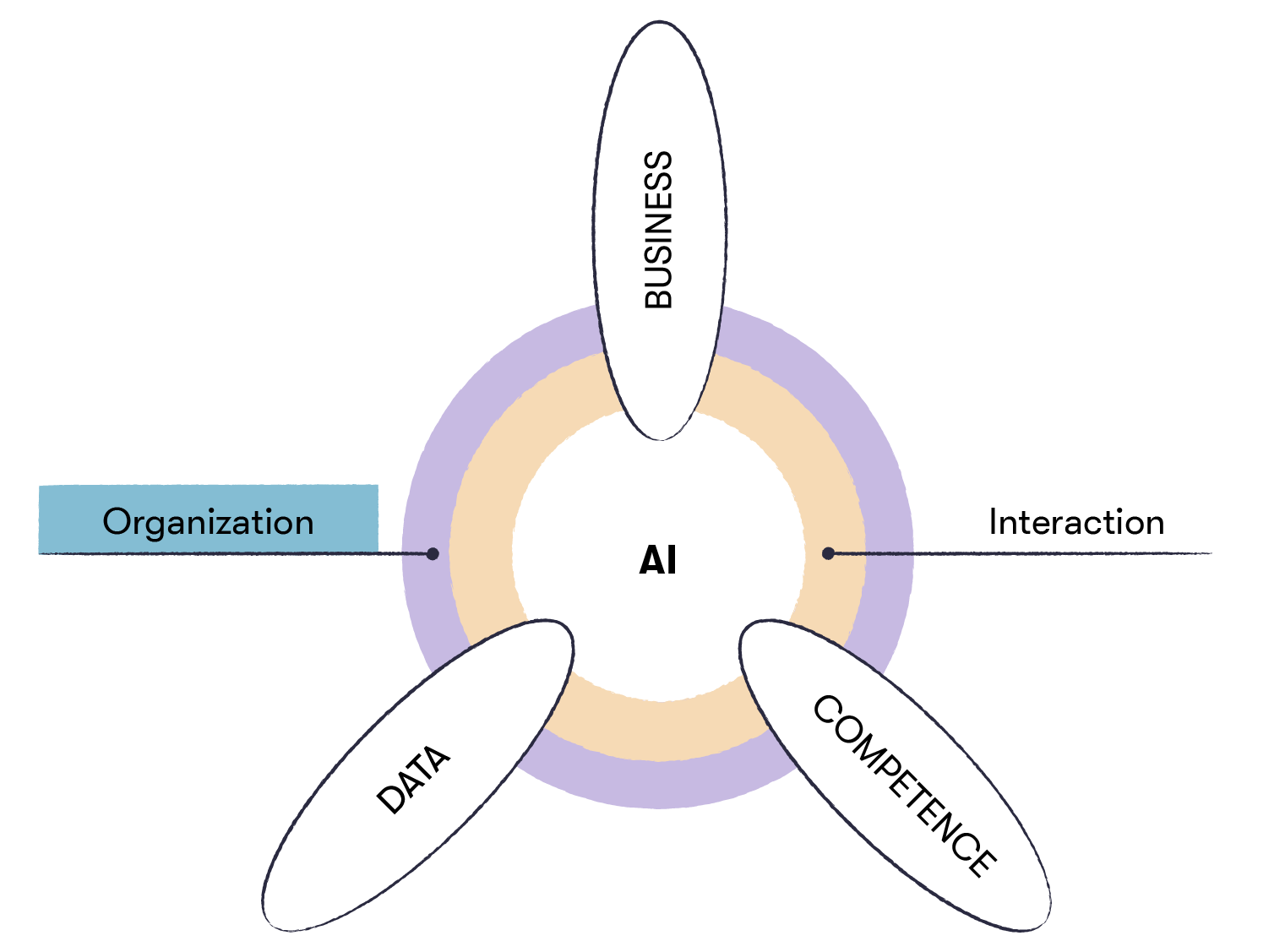
Managing AI projects and development work means continuous change management. As we’ll see with the industry-specific AI solutions presented later in this chapter, there is no single right way to structure an organization when it comes to AI development.
An organization is a balance between hierarchical structures and self-guided ways of working, both of which have their advantages and disadvantages (see chart below). The challenge is to identify, design, and implement a model that supports the strengths of both approaches.
Hierarchical structures support centralized management, while decentralized ones support self-guided.
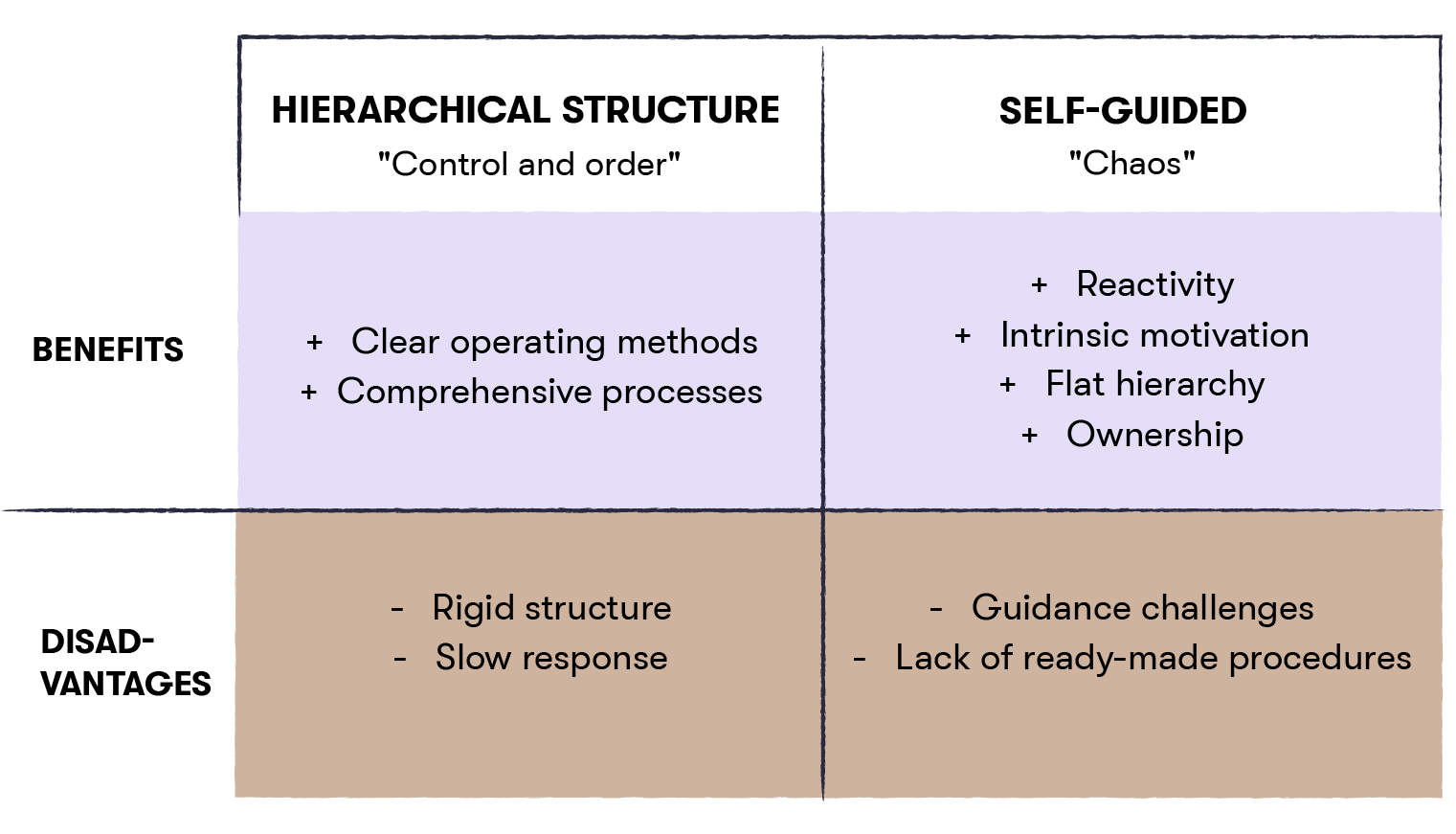
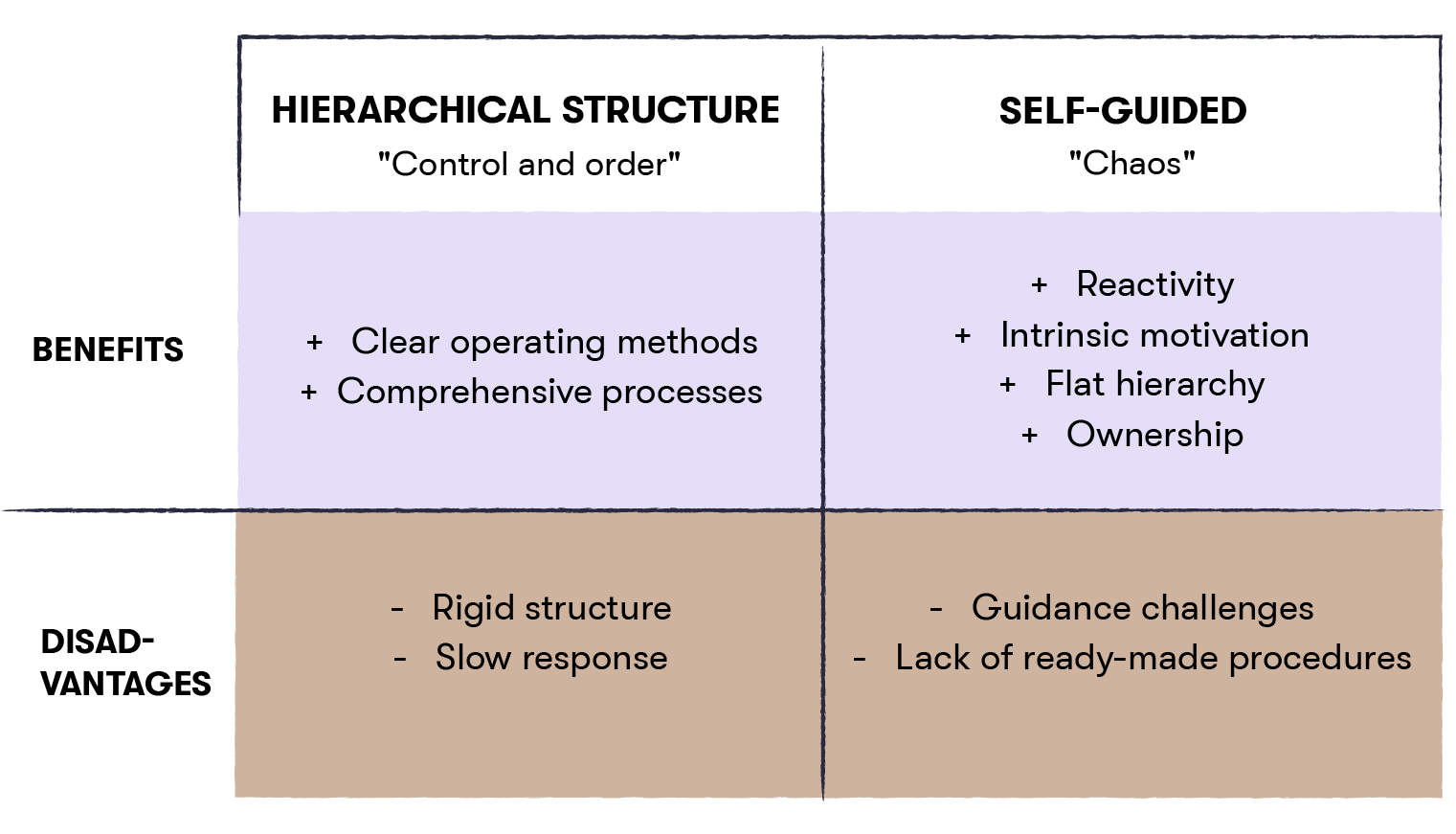
It’s ideal to try and find a model that suits your company and its culture.
Some degree of centralization helps promote the development of competence. In a more decentralized model, internal networks can help achieve the same effect.
Reading recommendation
Harvard Business Review’s article, “Building the AI-Powered Organization” in the “Organizing for Scale” section, illustrates the dynamics of decentralization and centralization.
Tools for an agile organization
Whether an organization’s model is centralized or decentralized, there are well-established ways of organizing teams and activities. There are a few principles, practices, and tools that work and some of these will be covered here.
Virtual teams are usually decentralized and communicate digitally. However, virtual teams are not the only route to success. Regardless of the organization type, it’s essential to find the right combination when it comes to the distribution of the budget, the allocation of people's work, and the flow of information between different stakeholders.
AI projects and development usually require technology and IT architecture changes that the IT department may not be able to implement in an agile way. The cure for this is the “dual IT solution”, where there is a software interface between new application development and traditional systems for necessary data transfer and use of server resources.
The use of a ready-made agile development framework promotes cooperation and communication between relevant parties, as well as working towards a common goal. There are many useful frameworks and tools available. An example of this is the scaled agile framework.
For AI development, it’s worth learning about the development of digital services, where cross-disciplinary teams and service design are commonplace. The development of AI is multidisciplinary and always requires several different perspectives. The composition of the team should also adapt to the different phases of the development project.
Interaction
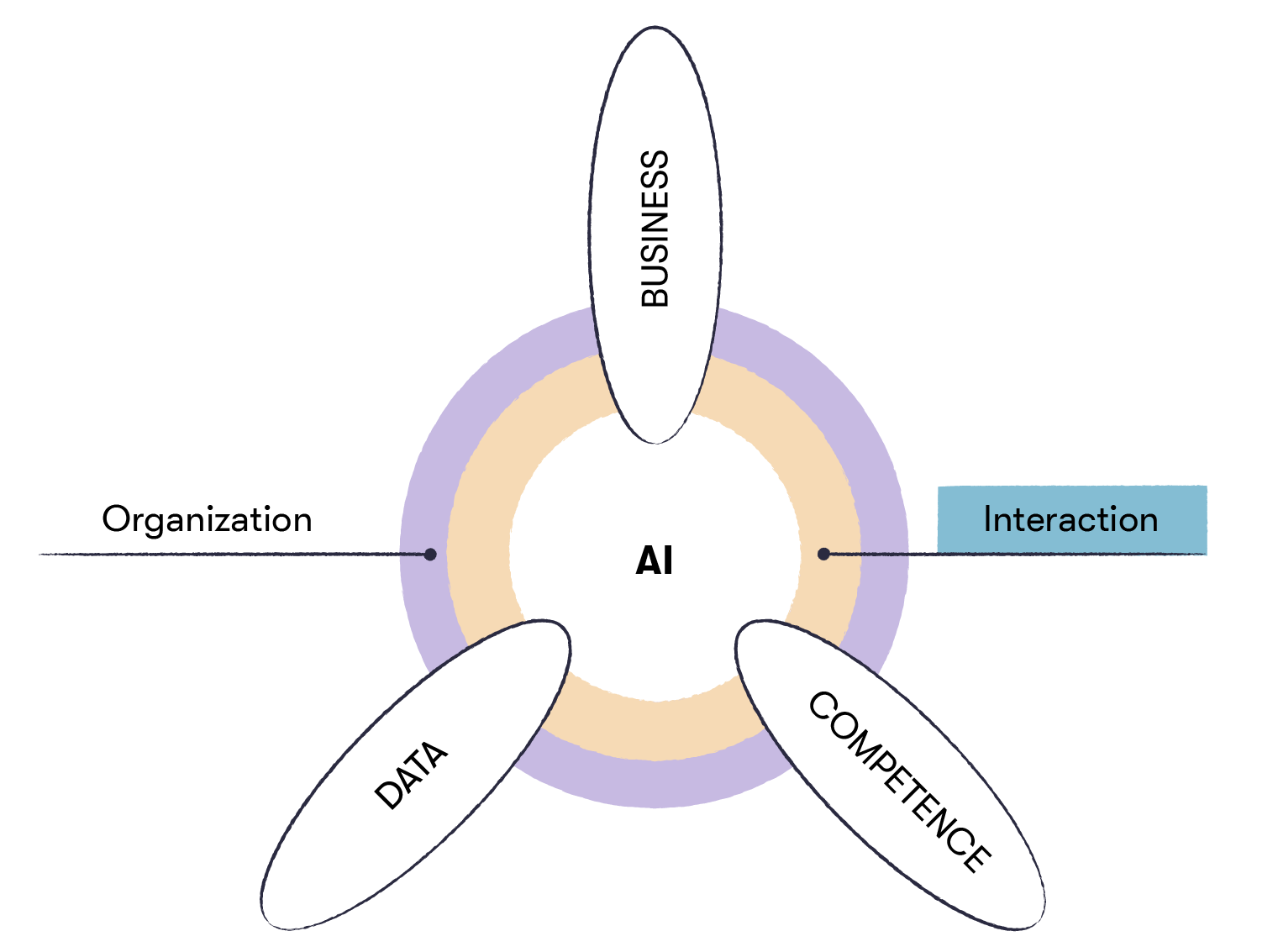
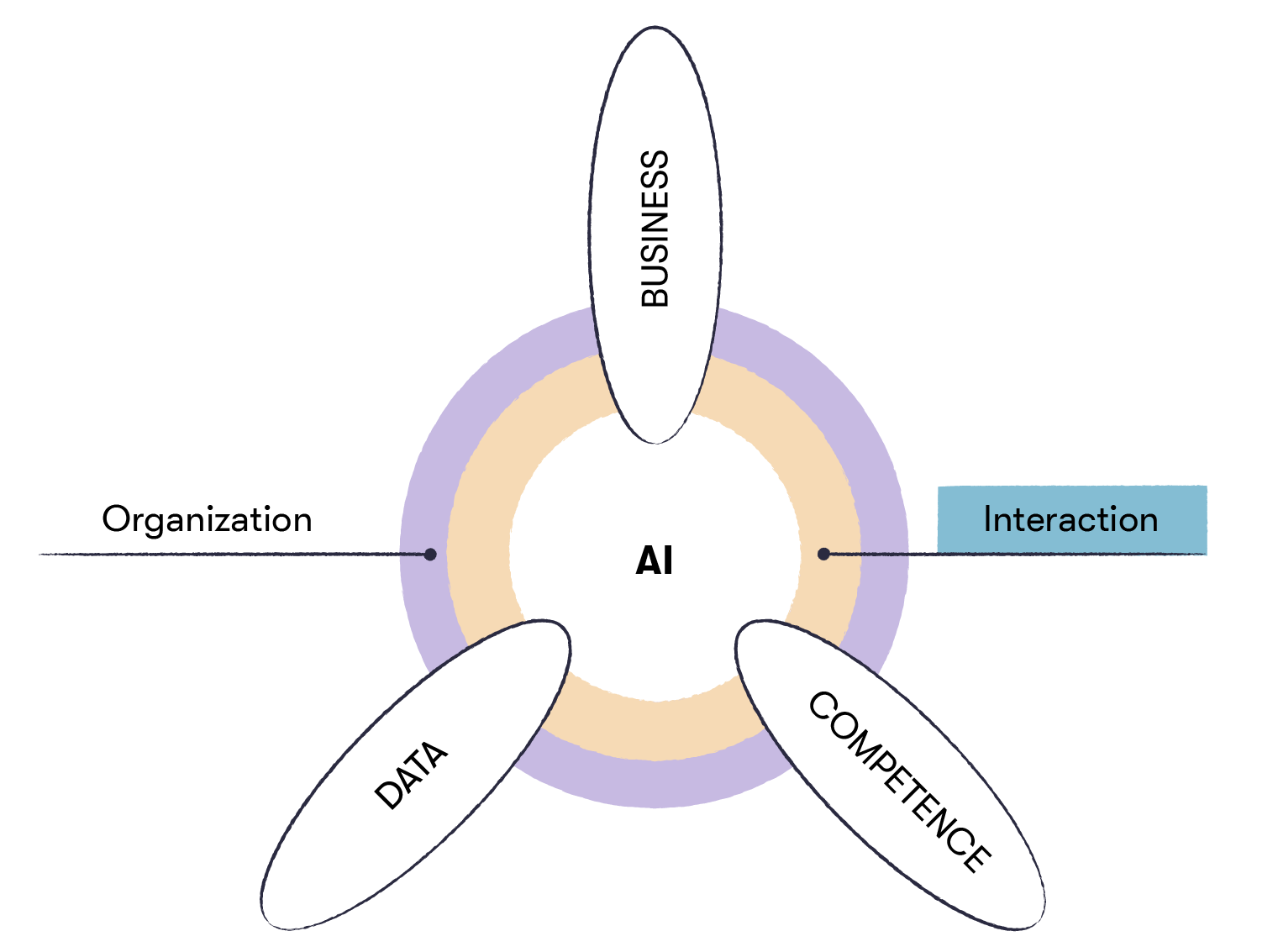
In AI projects, interaction is often about managing expectations.
AI projects are people-centered projects. Indeed, deficiencies in communication, building common understanding, and change management are the most common reasons where AI projects fail – not the technology itself.
In the most successful projects, the teams responsible for AI development (technology) and exploitation (business) spend the time required to reach a clear common understanding of goals and metrics.
Before starting a project, it’s only possible to create a superficial level of consensus. If participants do not deepen this during the project, the result can be a situation where goals and metrics mean different things to different participants. The time spent on careful goal definition and interaction therefore pays for itself many times over.
The power of examples
Often the same AI technology is suitable for many different uses. It’s therefore worth inviting people from different organizations to talk about their own AI solutions and applications. Concrete examples can inspire people to think about how a similar solution could be used for their own activities.
One of the most effective ways to increase AI interest and knowledge is through internal events, where your own people talk about their development projects and their successes and potential failures. One day a year is too rare for this purpose. We recommend holding regular events on a quarterly or monthly basis, or even more frequently. As with staff training in general, systematic and long-term sharing of experiences produces the best results.
In internal and inter-organizational events, demos are the most effective means of communication. Technically, simple demo versions tend to work better than just slideshows.
Agile development of an AI solution
There is a company that builds AI solutions based on an agile development model using two-week cycles. At the end of each development sprint, the developers organize a demo for a group of business representatives where they present the progress, discuss the outputs, and explain what happens next.
Common language
AI projects are often on the edge of something new, and this is why it’s important to clarify the concepts in order to talk about them. You should also use concepts everyone can understand.
A simple way to ensure a common language and understanding is to visualize the stages of the project by answering the questions: who, what, how, and when?
In AI projects, the organizing and interaction do not depend too much on the industry or the size of the company – instead, they are based more on the company’s way of operating. These key factors should be kept in mind when planning development projects, as they are easily forgotten when implementing.
Sign up to solve exercises
In the first chapter, you learned about the following:
How artificial intelligence is already a part of our everyday lives
Examples of natural language and machine vision artificial intelligence applications as well as numerical artificial intelligence solutions
Basic information on artificial intelligence and machine learning
How to identify three categories of machine learning: supervised, unsupervised, and reinforcement learning
How artificial intelligence is currently narrow, and not yet the self-conscious, general artificial intelligence of science fiction
The basic pillars of artificial intelligence utilization: business orientation, competence, and data
Managing artificial intelligence projects involves continuous change management where organization and interaction are crucial factors