In this Chapter, we will look at how to identify use cases for AI in business environments and how to assess their feasibility.
1) Identifying use cases
Several surveys of companies show that one of the biggest obstacles to using AI is finding suitable use cases, despite the fact that the internet is flooded with examples from almost every industry. The reason for this is that the operating principles and conditions that make for a successful artificial intelligence project are unclear. By studying case examples in more detail, it becomes easier to identify good use cases in your own business area and beyond.
The following checklist can be used as a guide for evaluating AI use cases.
A good use case checklist
1. The use case has a business need and an owner. This is required for a potential project to receive sufficient resources and a mandate to move forward.
2. The use case’s benefits are measurable or well justified. The benefit doesn’t have to be financial; it can be qualitative, for example improved job satisfaction.
3. The use case is unambiguous and detailed. Current machine learning-based AI solutions are good for doing one thing at a time (as explained in Chapter 1).
4. There must be sufficient quality data available, or it must be collectable. If the availability of data sets is limited, then the potential to use generative AI to create synthetic data should be assessed. Also, during the data collection or synthetic data generation process, the importance of ethical and legal guidelines should be highlighted.
5. The solution around the use case must also be practical (a production solution). Its implementation should therefore not require unrealistic investments or process changes.
6. The practicality of solutions should consider not just financial investments, but also the ethical implications of AI/GenAI, including biases and the potential for generating misleading information.
The use case may be solvable without using AI. For example, in an online customer support chat a person could answer questions instead of using AI methods. In this case, the strengths and weaknesses of the alternatives must be weighed up in the short and long term.
If there are several identified use cases and they all pass the previous checklist, they must be prioritized. The following simple evaluation matrix can be used as an aid.
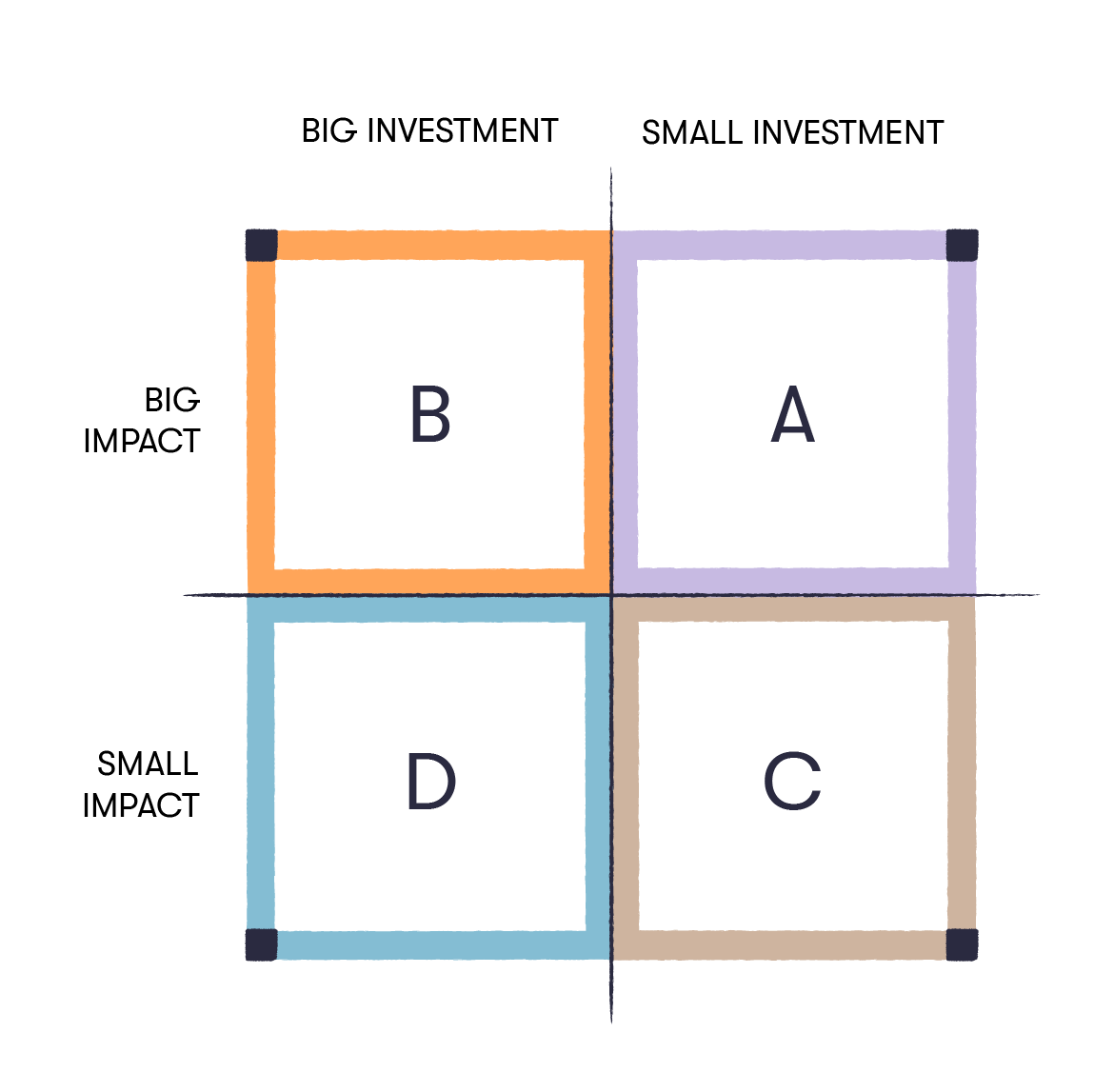
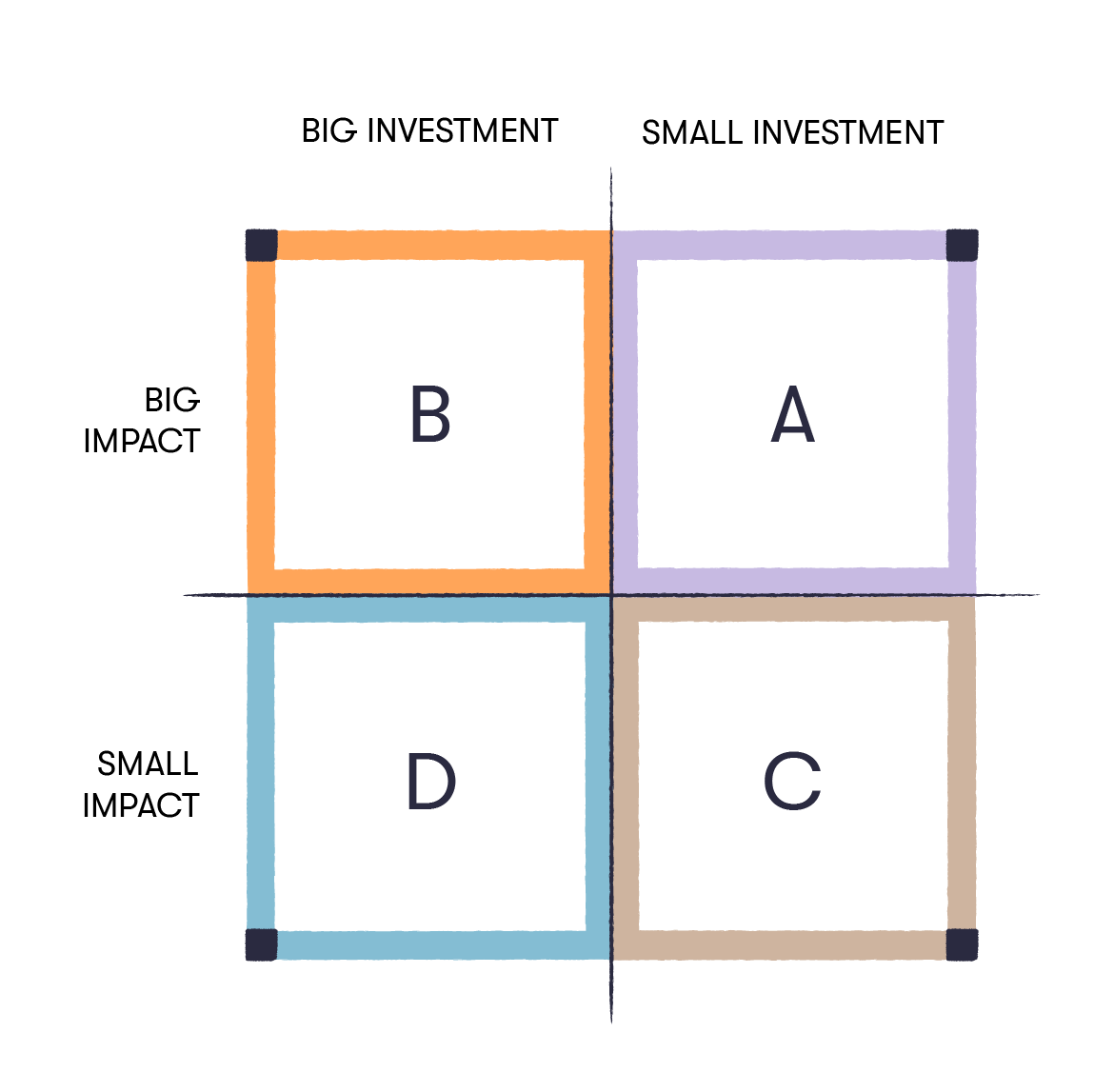
In the top-right corner of the matrix (A) we record use cases that have a large impact without large inputs. More often, cases are located in the lower-left corner (D), where a large investment produces modest benefits.
Generative AI based solutions may lead to creation of new business models or radically transform customer experiences, which may not have immediate, direct impacts but offer significant long-term value. Besides purely considering the impact and input dimensions, it is also important to consider risk as well as ethical issues, including data privacy, copyright issues, and potential misuse of generated content. Use cases with higher ethical risks require more thorough governance and oversight, which could impact their feasibility and public acceptance.
Sign up to solve exercises
Comparing implementation options
Once the preliminary assessment and prioritization round for use cases has been completed, it’s time to look at possible implementation options.
Here is an example of a comparison of implementation options:
There is a finished AI product on the market that is suitable for solving the use case.
There is a semi-finished AI product on the market that can be further trained with data specific to the use case.
There are no AI products on the market that are suitable for the use case. In this case, a customized AI project is the only option.
Identifying cases of AI use is an important step, but often it isn't enough. The broader context in which a possible AI solution would work must be taken into account. For that, you can use an AI canvas.
2) The AI canvas
Overall, whether or not an AI project will be successful is not self-evident. Not all AI projects have achieved their goals even if the solutions produced were very successful from a technical standpoint. Indeed, the reason for failures is related to factors beyond AI technology that haven't received sufficient attention.
An AI canvas helps you look at a project from multiple perspectives. In addition to the technical conditions, your canvas will take into account the business factors and tasks for the designed solution.
There are different types of AI canvas. In this course, we’re using the one shown in the figure below.
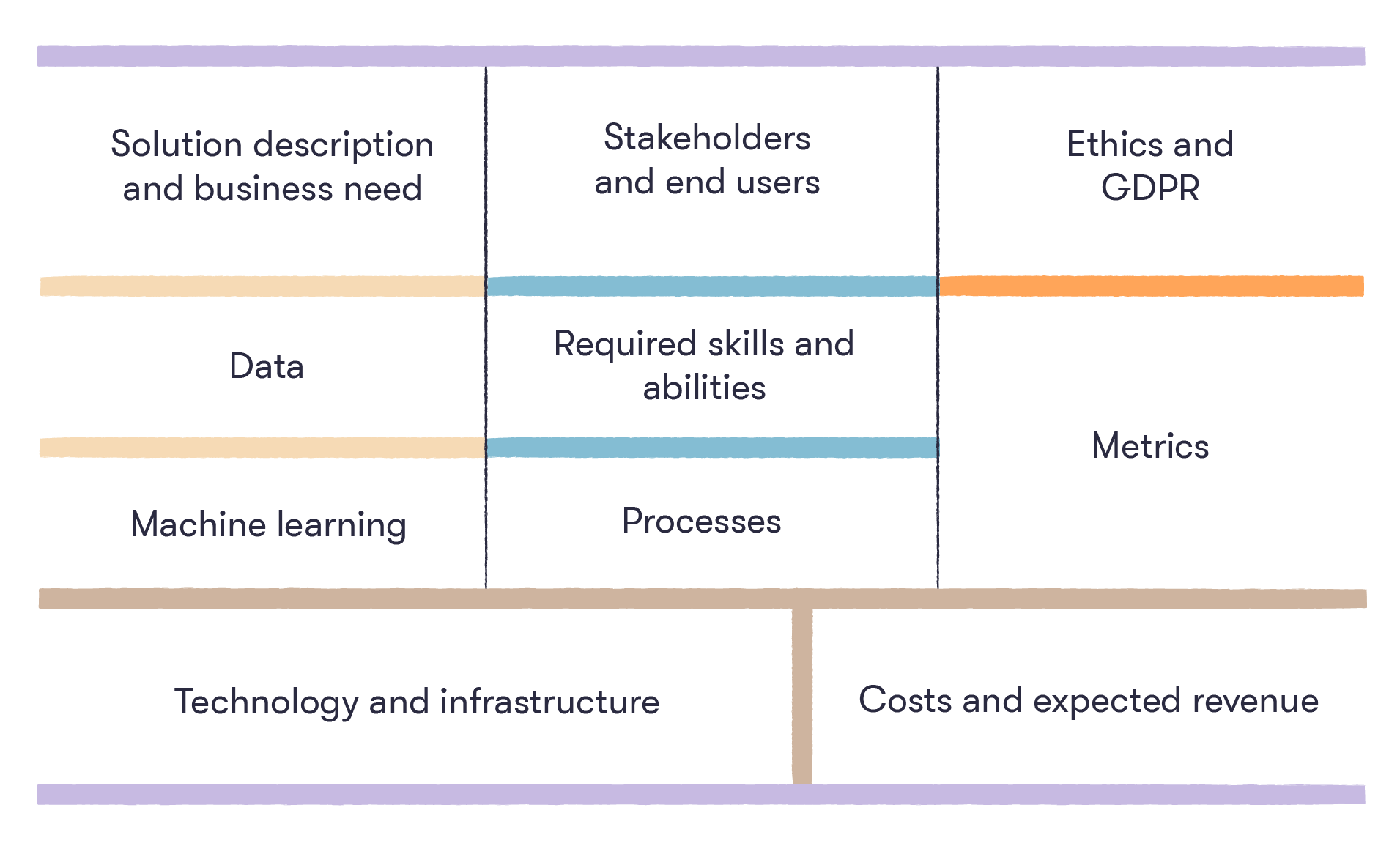
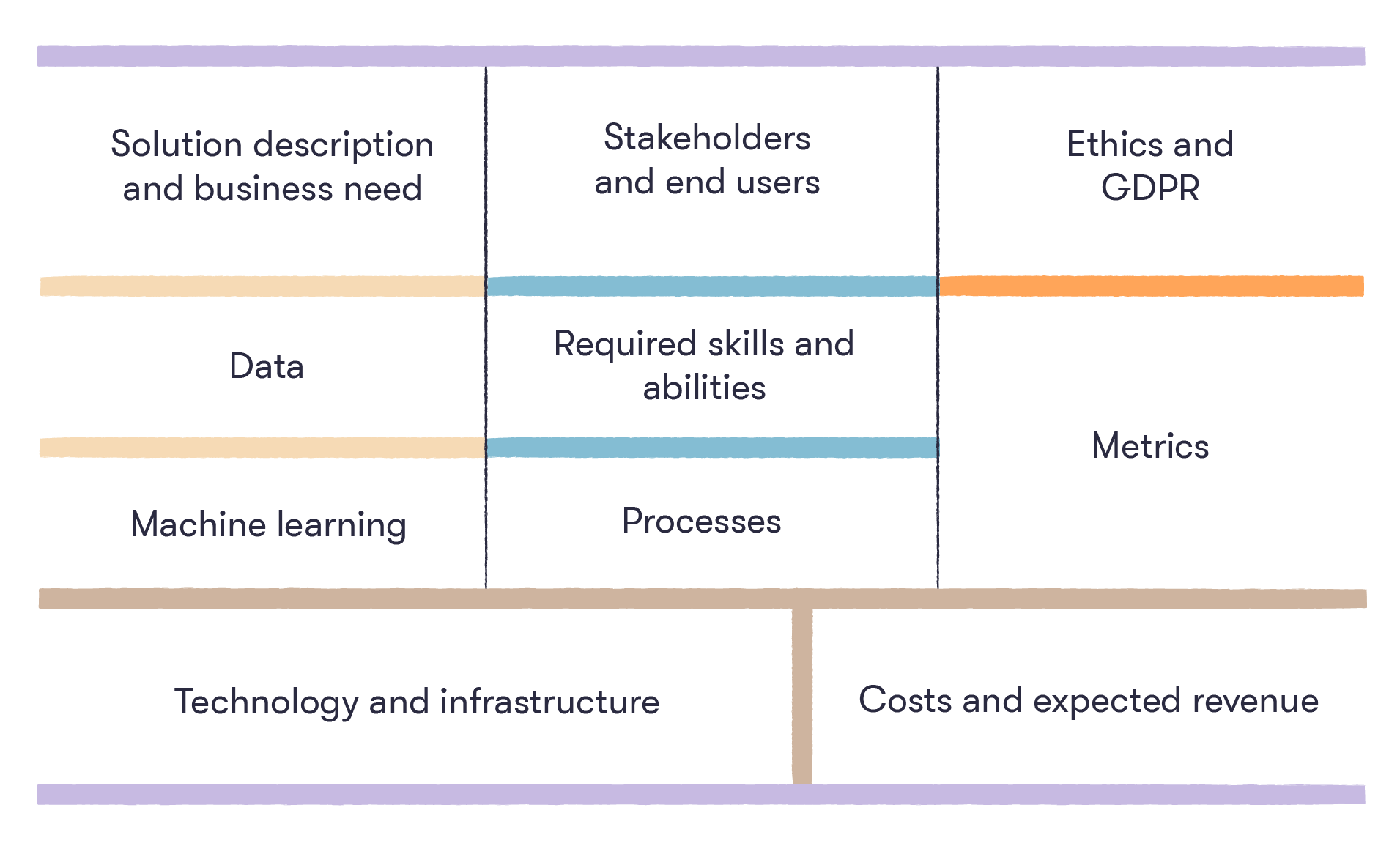
The AI canvas consists of ten areas that affect the implementation and use of an artificial intelligence solution. The goal is to describe each planned use case in its own canvas, point by point. If you think some of the issues are particularly critical to the use case being described, address them first.
The structure of the canvas isn't set in stone and the fields can be combined. What is essential is that key issues can be considered with sufficient precision.
Areas of the AI canvas
Next, let's look at the components of an AI canvas – some of them overlap with the checklist above. In this way, the use of a checklist brings pre-considered content into the AI canvas.
Solution description and business need
The clearest way to start using a canvas is to describe the planned solution, including what problem it solves, whom it affects, and how it works in general. This field should tell the reader what the solution is and why it’s necessary.
Even if there’s a clear business need for the solution, it may not support the company’s strategy. In this case, special attention should be paid to whether sufficient resources and authorization are available for the project to proceed.
Return on investment
In business, investments are usually meant to be productive. There are three ways to look at an investment: returns, costs, and risks. This model also applies to the design of AI solutions. When assessing risks, special attention should be paid to the resources required, the availability and quality of data, and the expertise needed.
A review of a planned solution can begin by evaluating its benefits. These could be for example saved working time, increased sales, lower costs, energy savings, or reduced damage. In the optimal situation, the benefits can be measured and described numerically. As previously mentioned, Generative AI based solutions may lead to creation of new business models or radically transform customer experiences, which may not have immediate, direct impacts but offer significant long-term value.
CFO's thoughts
Consider the following hypothetical situation from a chief financial officer’s point of view: if the number of defective deliveries decreases by 10%, the company would save EUR 300,000 annually and EUR 1,500,000 over five years. Perhaps AI could be used to help find faulty delivery items?
However, making an accurate return calculation is often difficult or even impossible. It’s therefore essential to understand the true magnitude of the expected returns.
The other side of the investment calculation is a cost estimation – all development and operational cost factors must be taken into account. Let's look at this using two examples.
Bid pricing
Imagine that a company implements an AI solution for bid pricing. All the necessary data, software, and technical infrastructure for implementing the solution are in place and available.
The majority of the project costs consist of design and implementation work, which is partly purchased from an external supplier. Internal costs arise from time spent on the project and the training of salespeople to use the solution, and/or business process redesign and implementation. There might be additional costs incurred related to customer acquisition e.g. marketing costs.
Identifying defective products
Imagine a company that is going to implement an AI solution to monitor products along the production line and automatically detect defective products. To do this, the company must acquire camera equipment for the production line, change the operating logic of the production line, collect data, build data pipelines, and have the necessary data storage infrastructure. In this context, a data pipeline means all the components required for the transfer, modification, and storage of data used in an artificial intelligence solution.
In addition, the company must purchase a separate server for AI at the factory and make software changes to the production line software. As there is no expertise for this in the company, the company must procure the entire solution from other companies.
In the second example, there are significantly more cost elements and the project is clearly higher in both cost and risk.
Like the calculation of revenues, accurately estimating costs in advance isn’t always possible. In this case, it's essential to estimate the correct cost ranges.
Sometimes you might hear that the potential return of a planned AI project should be as high as possible, for example that the expected return should be 10 times higher than the investment itself.
Sign up to solve exercises
If it’s difficult to make an investment calculation, the following four fields can be used to help you position the planned solution based on the best estimates. The four fields also help to discuss investment projects with various stakeholders.
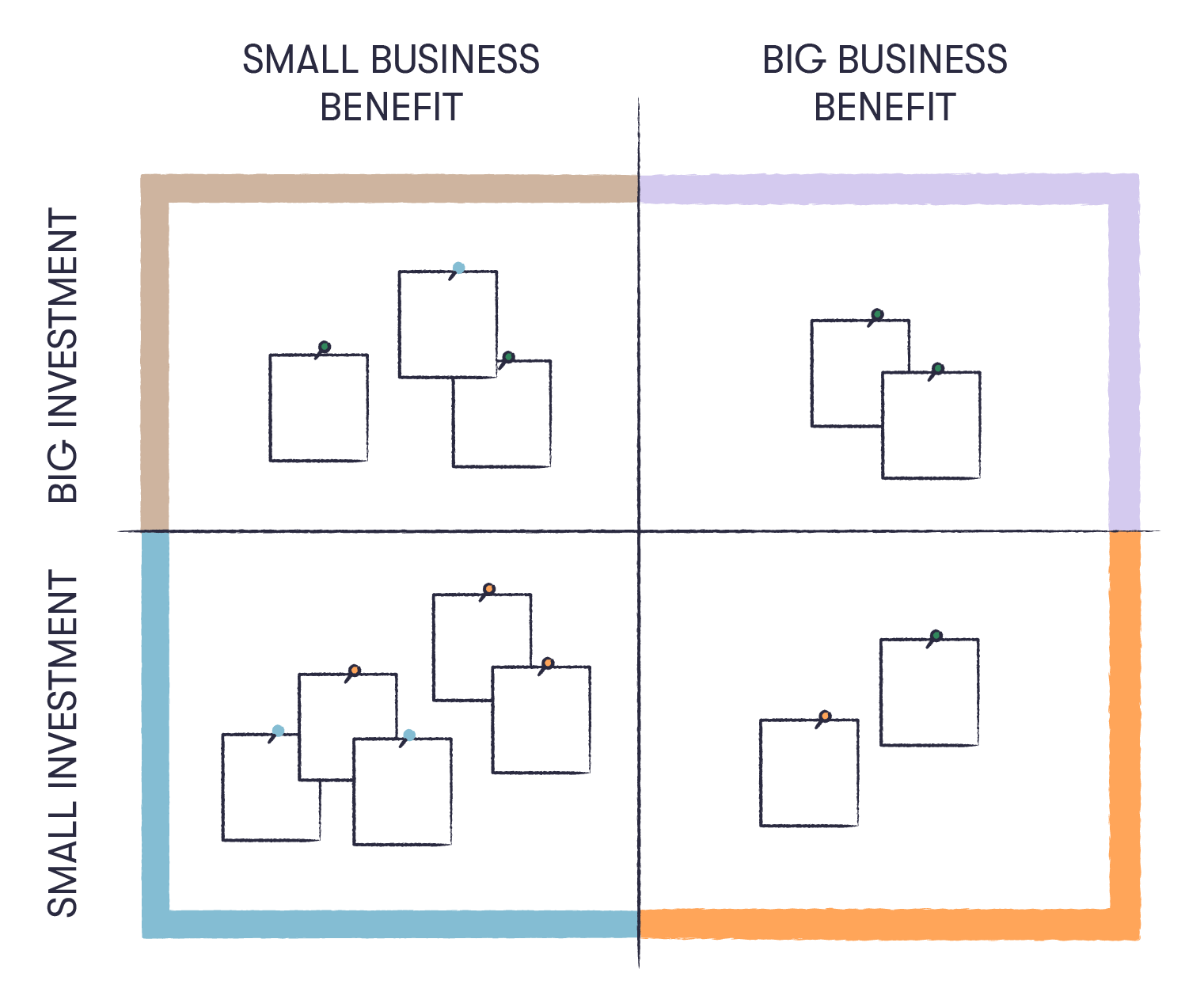
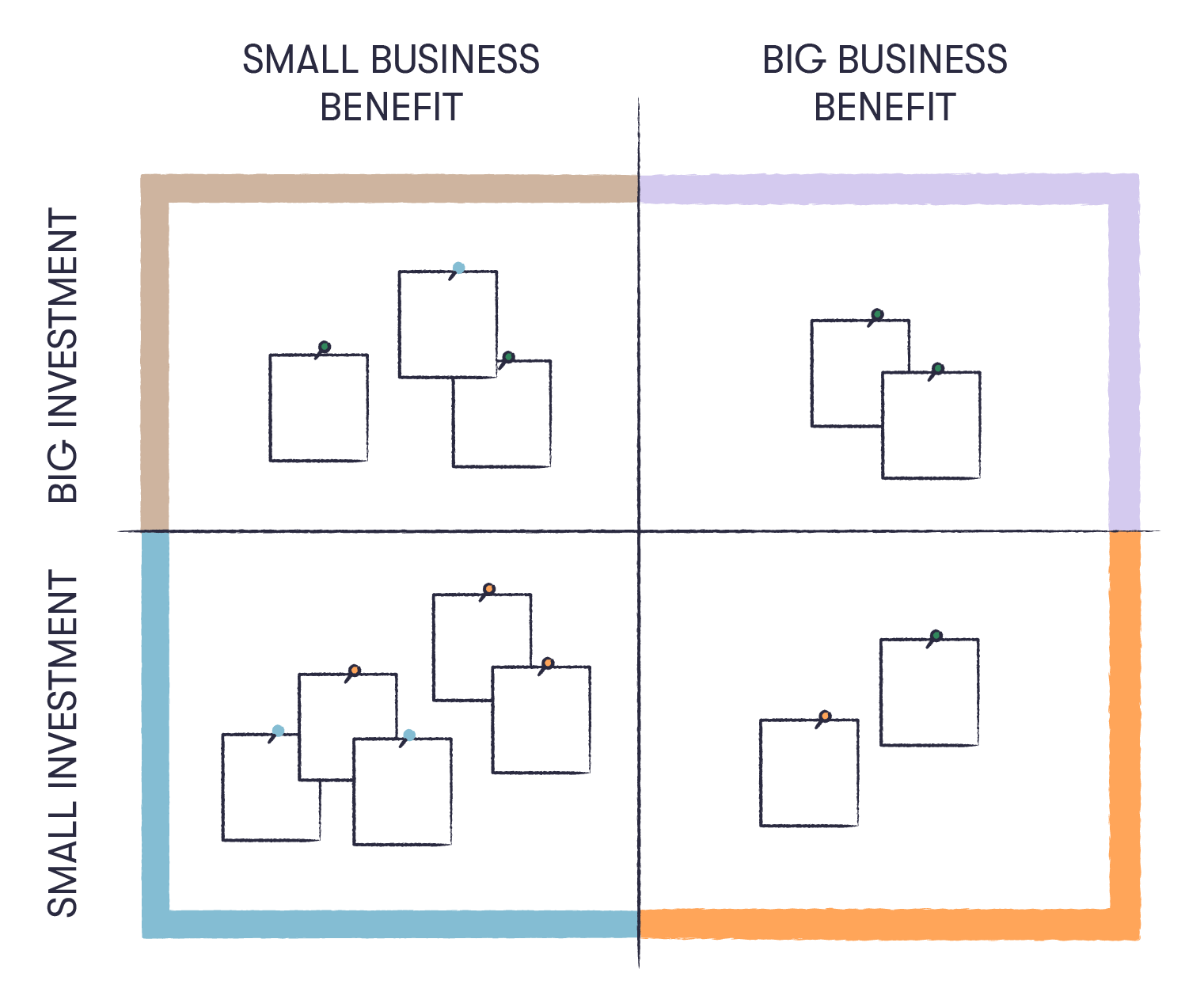
Investment return matrix
What if the investment isn't measured directly in financial terms?
When assessing the usefulness of an AI solution, the figures in the investment calculation alone aren't always enough. Even if it's possible to make accurate investment calculations, qualitative factors may weigh more than monetary ones. Below are a few examples of this.
The investment is related to the achievement of a strategic goal, such as the promotion of sustainable development.
The investment increases job satisfaction, for example by reducing manual and routine work steps.
The investment can be used to improve the quality of a product or service and, as a result, customer satisfaction.
Many of the GenAI projects being implemented lead to productivity improvements in business processes or tasks of knowledge workers. Some of the productivity improvements may be due to part or full automation, which may impact the content of existing tasks performed by workers.
In these cases, the investment calculation or a more rigorous cost-benefit analysis will still have a place in the decision on whether to implement the AI solution.
Metrics
The metrics field is used to define the AI solution’s goals and how success is measured. There are two types of metrics: numerical and qualitative.
Numerical indicators include things like cost savings, an increase in sales, an increase in production volumes, an improvement in lead times, and a reduction in accidents.
Qualitative metrics aren't based on unambiguous numerical values. For example, user feedback or customer interviews provide qualitative data. A qualitative indicator could also be the ability of a solution to promote sustainable development goals in a company, or improvement in customer experience, for example.
Thus, metrics will help to assess how successful an implemented solution has been and how it should be developed in the future.
Stakeholders
During a solution’s design phase, the key stakeholders whose input is needed to implement it and whose work will be affected by it should be identified. Stakeholders vary from project to project, so no universal list can be created. However, here are a few examples that are repeated in most projects:
Project owner and sponsor. They are responsible for promoting the planned solution and, among other things, using the AI canvas.
Business executives who are well acquainted with the business being addressed and its laws.
An IT organization that is typically responsible for implementing, developing, and maintaining a solution.
Potential supplier partners (AI, data, software development, etc.) with whom the solution will be implemented.
The end users or groups whose work is affected by the solution.
Experience has shown that if a project has no business owner or user group, it's less likely to succeed.
Skills required
The know-how required for AI projects varies between different solutions. Typically, projects have the following roles: product owner, data engineer, data scientist, and project manager. With the advent of GenAI, the need for new roles have come up. Some of these roles are prompt designer/ engineer, prompt QA. The same person can act in multiple roles as needed. Because communication and interaction are important, the project team must have expertise in both internal and, where appropriate, external communication.
Depending on the project, roles other than those mentioned above are also needed. For example, if the end product is a custom mobile application reinforced with AI, the project will also require a software developer. If, on the other hand, the output of the project is a visual report, expertise on reporting software is needed rather than a software developer. Where there are special needs related to the usability of the application, a service designer should be included.
The figure below illustrates the competence needs for a machine vision solution where the end result is a mobile application.
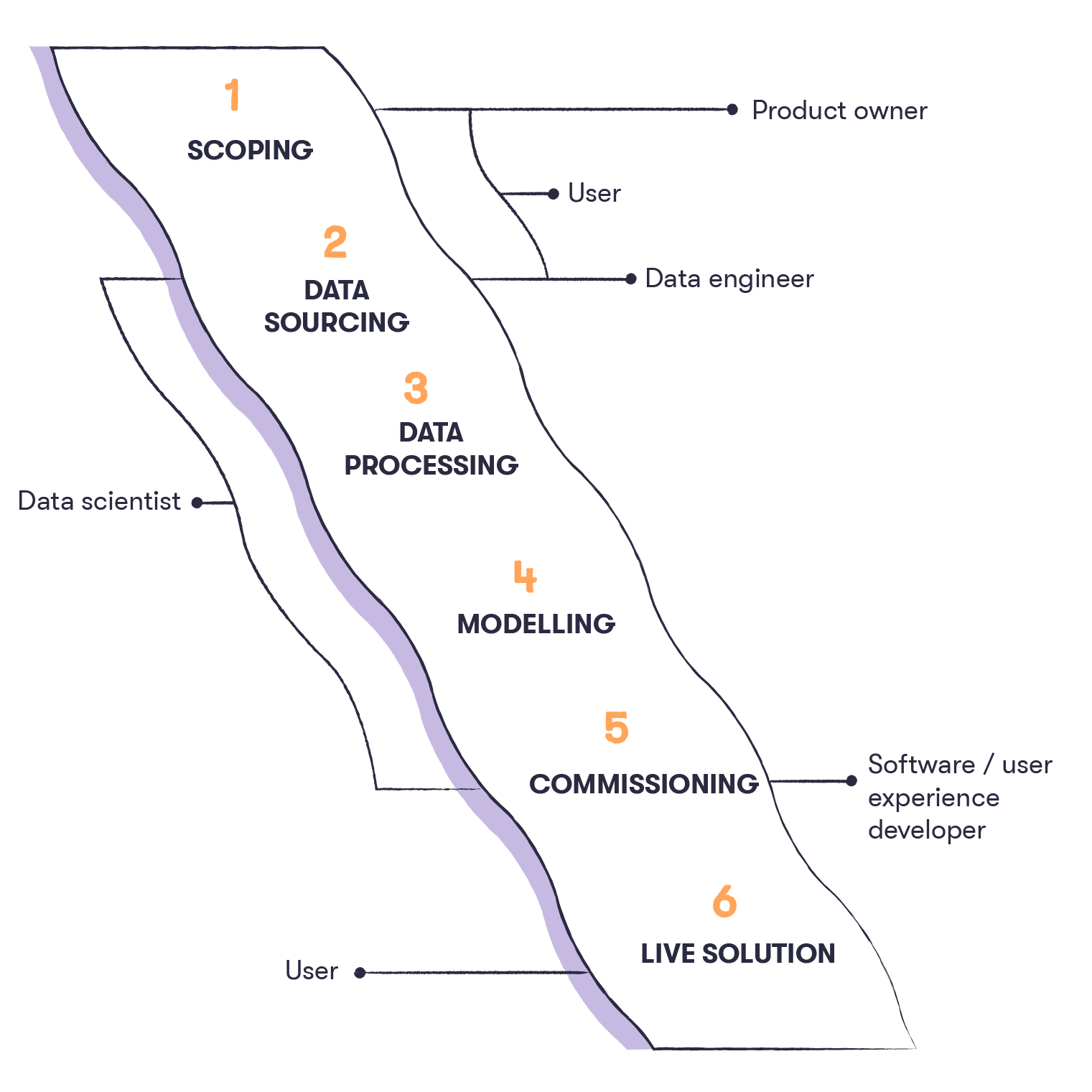
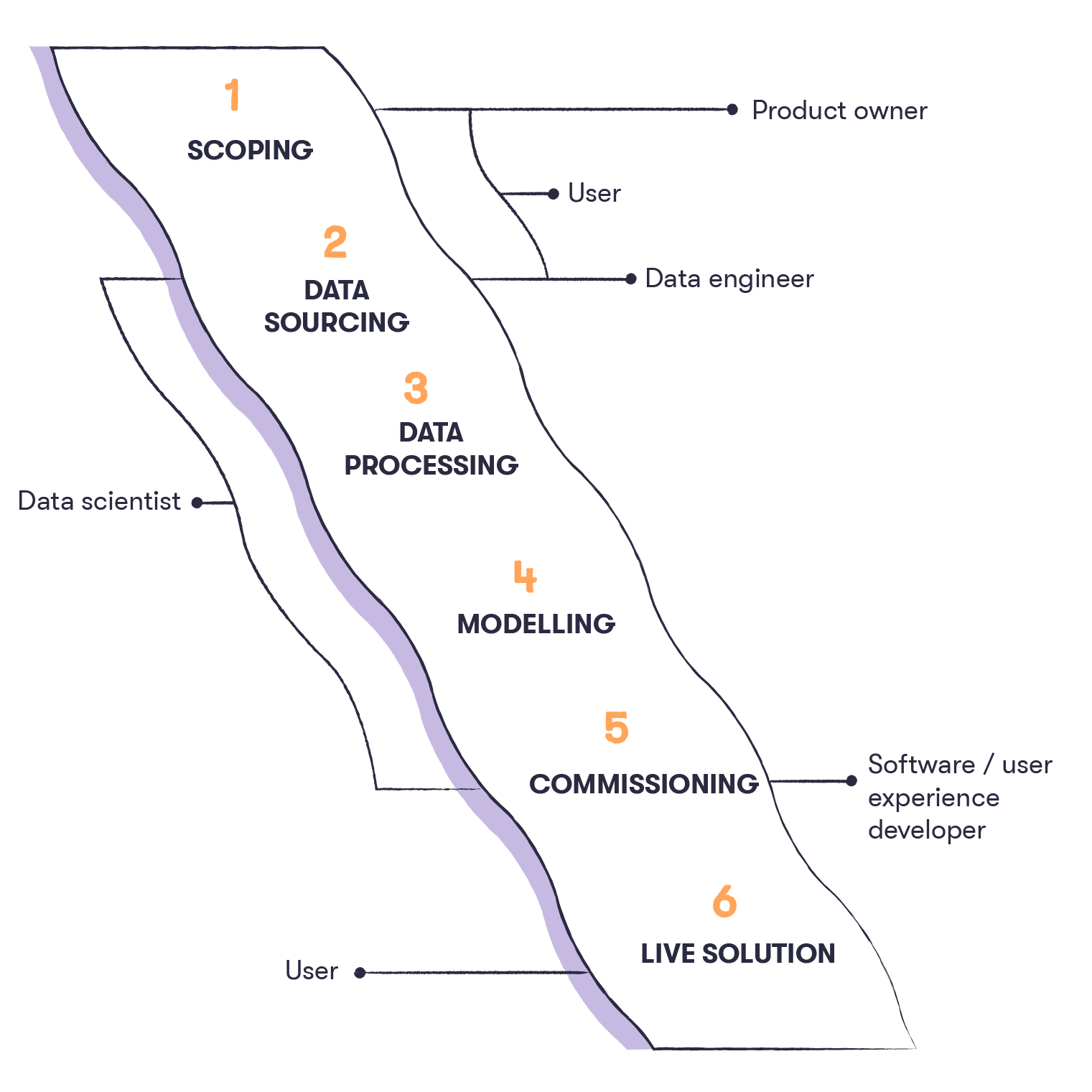
The typical roles in different phases of an artificial intelligence project
AI Canvas: Data
The most important element of an AI canvas is data, because without suitable data a solution cannot usually be implemented.
The following includes data issues that should be addressed during the project design phase:
What data is needed to implement the planned AI solution?
Does the required data already exist or must its collection be started?
Are there any known deficiencies in data quality that could affect the planned solution?
Are there any inherent biases in the data e.g. i) temporal bias due to data being collected at a certain time ii) social bias where the proxy of the variable (label) to be predicted may not well represent the true label of interest iii) population bias where the dataset may under or over represent some types of inputs.
Is the data available and are the associated data pipelines in place?
Is there enough data?
What’s the total cost of acquiring data?
Note: even if the data is available and the technical solution is possible, sometimes the problem may need not be solved as it may breach ethical behavior, AI laws, or may cause societal harm.
Quite often this field remains too general, which can lead to unexpected difficulties in acquiring data that is critical to the project.
When data is a bottleneck
One project manager stated the following about his project: “During the design phase we found that the required IoT data had been collected and stored in the cloud. When the project started, it was discovered that this data was only stored for two months at a time, which is too little. No one was aware of the quality of the data either, as it has not been previously analyzed or reported. Thus, the project encountered two problems: there was too little data and its quality was lower than expected.”
You should invest in clarifying the data. The availability, quality, quantity, and possible future changes affecting the data should be discussed with all relevant stakeholders.
When the format of the data changes during the project
Another project manager explained the consequences of an unexpected system change: “An AI solution was built for shift planning and was in pilot use. The next step was commercialization, during which it became clear that the data source the solution used, the ERP system, would change in six months, after which the solution would have to be re-trained with data according to the new structure.”
AI canvas and machine learning
While machine learning is at the heart of AI solutions, there is no need to delve deeply into it in an AI canvas. However, it's worth recalling the categories of machine learning we discussed in Chapter 1: supervised learning, unsupervised learning, and reinforcement learning.
Generative AI refers to models that are used to create or generate new content e.g. text, images, videos, audio etc., based on the patterns that the models learn during the training phase. Generative AI could fit in each of the categories of machine learning:
Supervised Learning - Generative AI model can be finetuned with specific training data to produce specific type of content. For example, it can be trained on a specific tone of voice to generate articles of a specific tonality.
Unsupervised Learning - Generative AI models can be trained on unlabeled data to generate new samples similar to the data distribution. This is done to improve performance of unsupervised models.
Reinforcement Learning - Used in scenarios where the generation process involves a sequence of decisions, such as generating dialogue in a conversational agent or actions in a game.
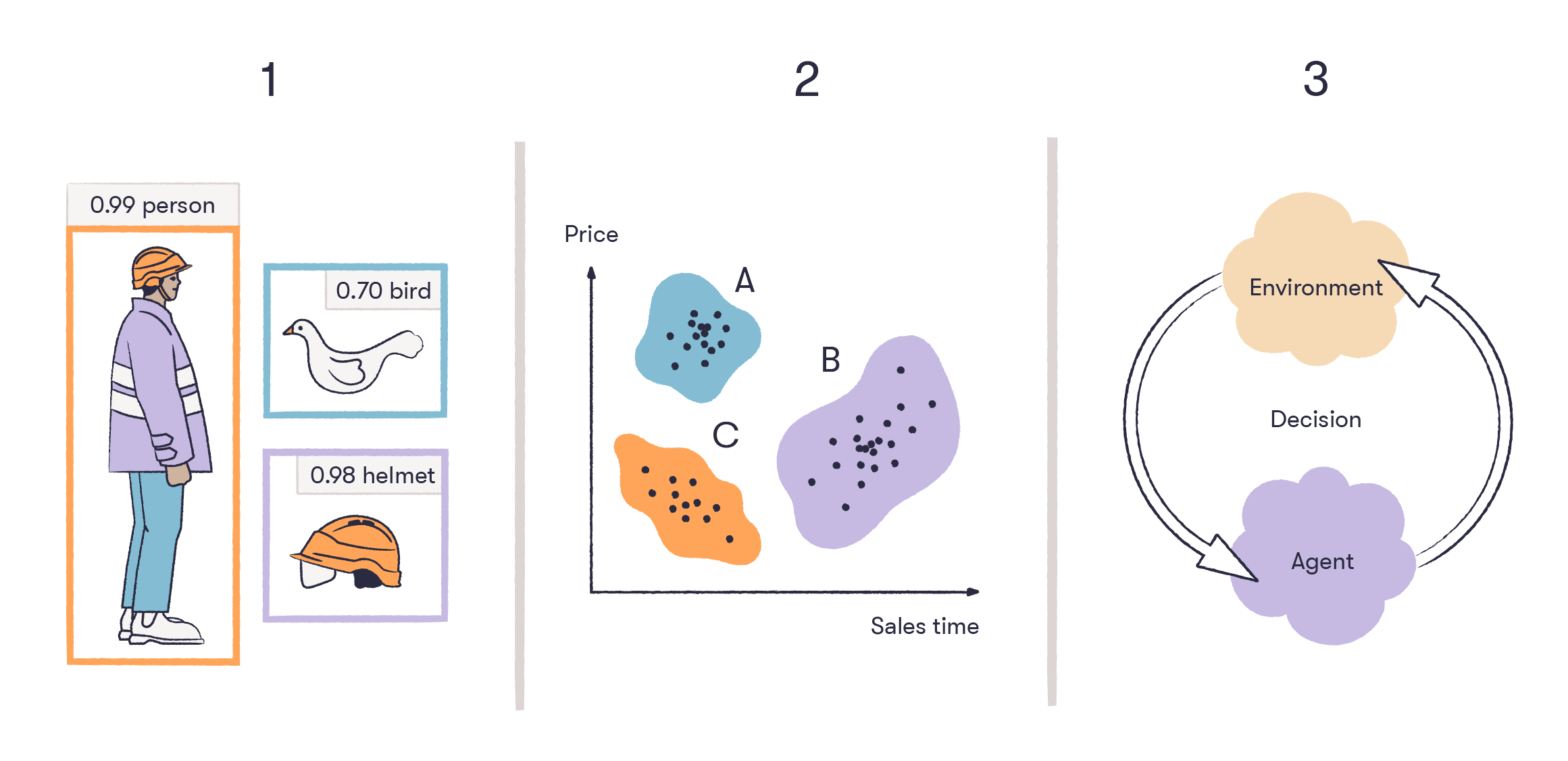
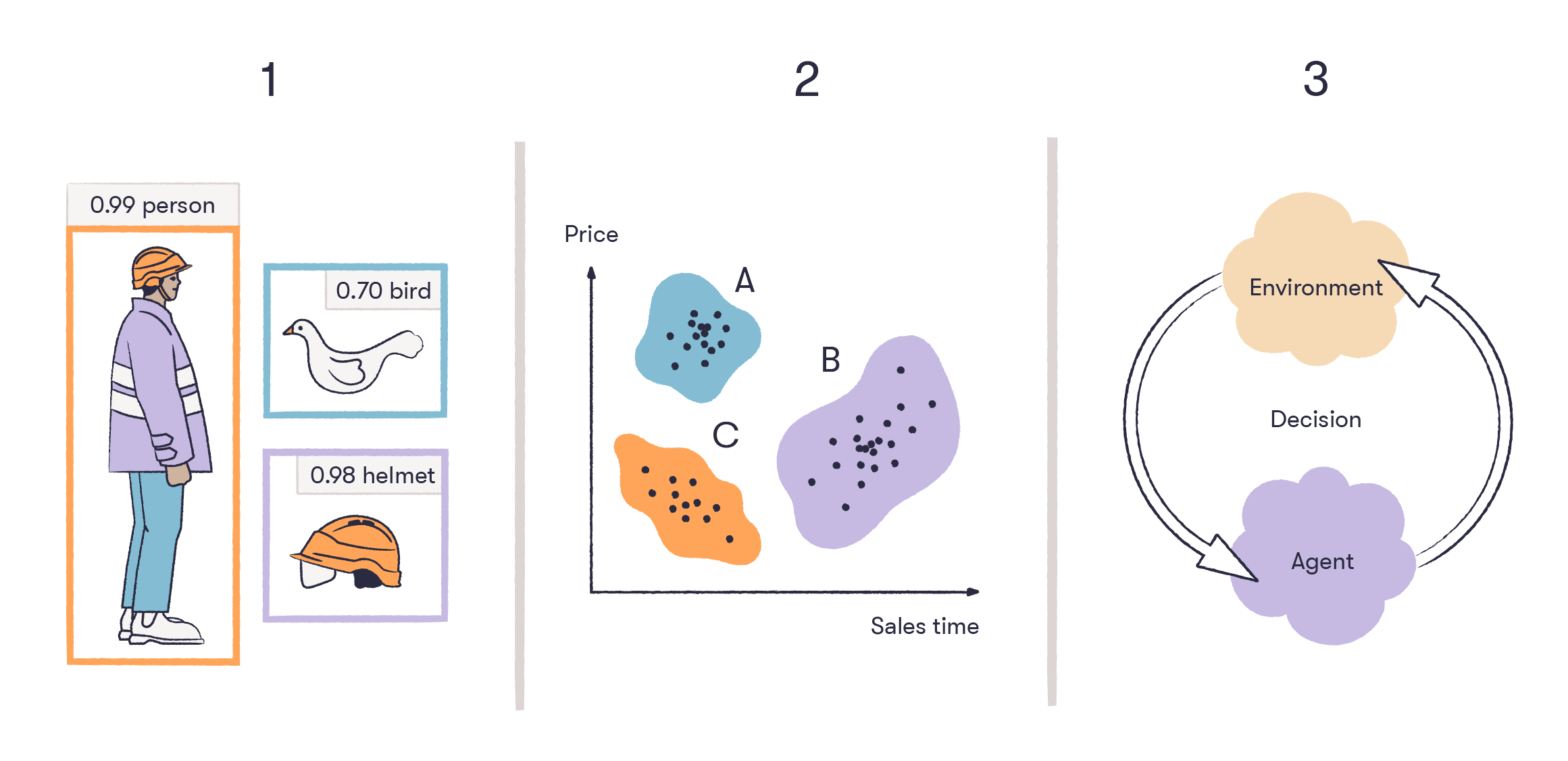
Categories of machine learning: 1) supervised learning, 2) unsupervised learning, 3) reinforcement learning
When designing any AI solution, you need to know which of the above categories the designed solution falls into. Each of them creates different solutions, the requirements of which differ in terms of know-how, the technologies used, and the data.
At this stage, it’s also possible to assess what other elements in the solution would be needed in addition to machine learning.
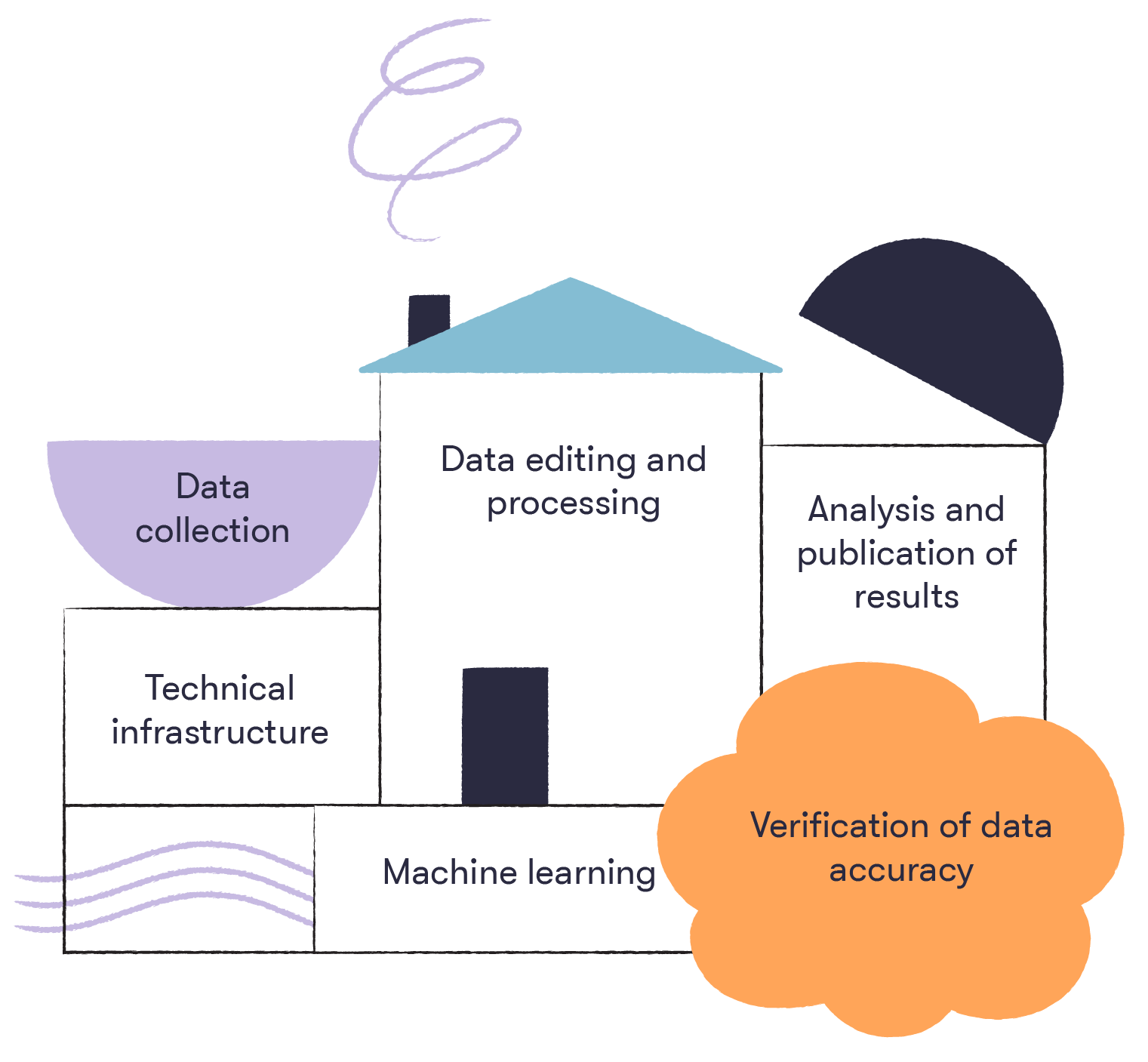
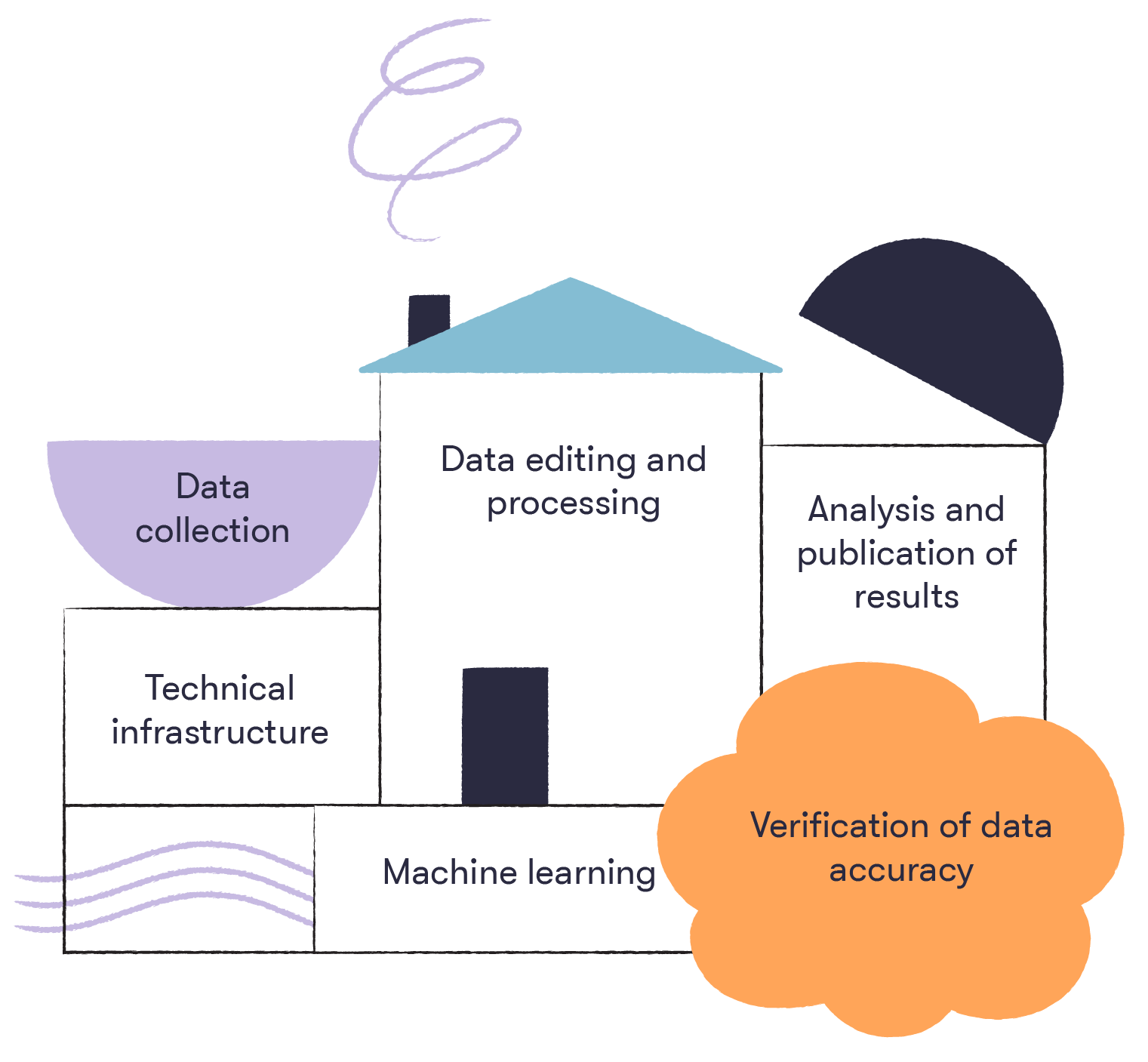
Machine learning is only a small part of an artificial intelligence solution. Inspiration to illustration from “Hidden Technical Debt in Machine Learning Systems,” D. Sculley et al.
The attached diagram illustrates how the elements surrounding machine learning can often be a much larger part of the solution than machine learning itself.
Software and technical infrastructure
What kind of software and technical infrastructure is needed to implement the planned solution?
The software design of the solution includes the tools to be used for data transfer, processing, storage, and visualization as well as the running of machine learning models and the analysis of results. Depending on the solution, the list may be long.
Technical infrastructure refers to the physical devices that produce data. These are typically cameras or Internet of Things (IoT) sensors. The transfer of data from devices to software requires protocols that must be clarified at this stage.
Design should not be limited to the needs of the development phase, but extended to future use too. This ensures that a successful proof of concept or pilot project is not prevented from going into production because the technical conditions for it are not in place.
Processes
It’s not uncommon for a company to implement a technically successful AI solution that is then not used. Often, the reason for this is that the solution hasn’t been integrated into the company’s processes and practices. The following two examples illustrate this problem.
Case example 1 (wrong way)
An insurance company implemented an AI solution for forecasting customer exit. The solution was quick to implement because the necessary data and software already existed. The results were also good. However, the solution remained unused because the insurance company lacked a process for putting it into practice. Customer loyalty was also not a measure of the performance of any of the business units so there was no motivation for using the solution.
Case example 2 (right way)
A large company implemented an AI solution that searched for the optimal price point for the bids made, optimizing the available margin and the probability of bid success. Getting the sales organization to trust and use the solution was critical to success. This was achieved by the vendors piloting the first version of the solution and then shifting focus to further development.
It's therefore important to clarify right from the design phase how the solution will be taken into use during the production phase.
Ethics and GDPR
Ethics and GDPR are new but important areas in the AI canvas. The ethics of AI and GDPR regulations must be taken into account, especially if the planned solution involves personal data or automated decision making.
GDPR (the EU’s General Data Protection Regulation) and its application may require legal knowledge and experience as violations can potentially be accompanied by significant sanctions. In the spring of 2021, fines of more than 270 million euros had already been imposed across Europe. In 2024, a huge fine of approx. 850 million euros was imposed on a large retailer in Nordics for GDPR violations. It's recommended that a GDPR expert be involved at the design stage of a project as it may be difficult for the average person to understand the coverage and application of legislation. The following example illustrates the problem.
Aha!
A company wanted to implement a solution based on machine vision, the images of which showed employees. The original idea was to programmatically blur people’s faces so that they could not be identified. However, according to the company’s GDPR expert, that would not be enough as a person can be identified by the way they move, their posture, or other external factors.
Ethics of AI and GDPR
Both of these areas are broad. You can find more information about them at the following links:
Ethical challenge – involving companies in the ethical use of artificial intelligence
Summary of the AI canvas
We have now gone through the content of the AI canvas step by step. The aim is to get as realistic a picture as possible of the conditions and risks for the success of the planned AI project before making an investment decision. The use of a canvas helps to separate the good ideas from the bad.